Generative AI for clearer communication: how it helps evaluate, adjust and clarify language
Fecha de la noticia: 05-02-2024
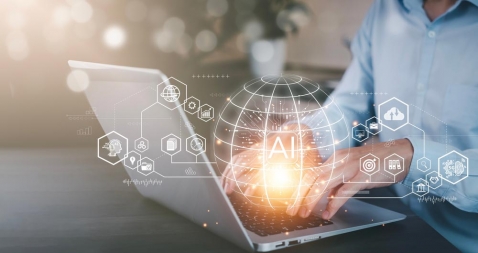
The alignment of artificial intelligence is a term established since the 1960s, according to which we orient the goals of intelligent systems in the exact direction of human values. The advent of generative models has brought this concept of alignment back into fashion, which becomes more urgent the more intelligence and autonomy systems show. However, no alignment is possible without a prior, consensual and precise definition of these values. The challenge today is to find enriching objectives where the application of AI has a positive and transformative effect on knowledge, social organisation and coexistence.
The right to understand
In this context, one of the main pillars of today's AI, language processing has been making valuable contributions to clear communication and, in particular, to clear language for years. Let us look at what these concepts are:
- Clear communication, as a discipline, aims to make information accessible and understandable for all people, using writing resources, but also visual, design, infographics, user experience and accessibility.
- Clear language focuses on the composition of texts, with techniques for presenting ideas directly and concisely, without stylistic excesses or omissions of key information.
Both concepts are closely linked to people's right to understand.
Before chatGPT: analytical approaches
Before the advent of generative AI and the popularisation of GPT capabilities, artificial intelligence was applied to plain language from an analytical point of view, with different classification and pattern search techniques. The main need then was for a system that could assess whether or not a text was understandable, but there was not yet the expectation that the same system could rewrite our text in a clearer way. Let's look at a couple of examples:
This is the case of Clara, an analytical AI system that is openly available in beta. Clara is a mixed system: on the one hand, it has learned which patterns characterise clear and unclear texts from the observation of a corpus of peers prepared by specialists in Clear Communication. On the other hand, it handles nine metrics designed by computational linguists to decide whether or not a text meets the minimum requirements for clarity, for example, the average number of words per sentence, the technicalities used or the frequency of connectors. Finally, Clara returns a percentage score to indicate whether the written text is more or less close to being clear text. This allows the user to correct the text according to Clara's indications and submit it for re-evaluation.
However, other analytical systems have established a different approach, such as Artext of course. Artext is more like a traditional text editor, where we can write our text and activate a series of revisions, such as participles, verb nominalisations or the use of negation. Artext highlights in colour words or expressions in our text and advises us in a side menu what we have to take into account when using them. The user can rewrite the text until, in the different revisions, the words and expressions marked in colour disappear.
Both Clara and Artext specialise in administrative and financial texts, with the aim of being of use mainly to public administration, financial institutions and other sources of difficult-to-understand texts that have an impact on citizens.
The generative revolution
Analytical AI tools are useful and very valuable if we want to evaluate a text over which we need to have more control. However, following the arrival of chatGPT in November 2022, users' expectations are set to rise even further. Not only do we need an evaluator, but we expect a translator, an automatic transformer of our text into a clearer version. We insert the original version of the text in the chat and, through a direct instruction called prompt, we ask it to transform it into a clearer and simpler text, understandable by anyone.
If we need more clarity, we only have to repeat the instruction and the text becomes simpler again before our eyes.
By using generative AI we are reducing cognitive effort, but we are also losing much of the control over the text. Most importantly, we will not know what modifications are being made and why, and we may incur the loss or alteration of information. If we want to increase control and keep track of the changes, deletions and additions that chatGPT makes to the text, we can use a plug-in such as EditGPT, available as an extension for Google Chrome, which allows us to keep track of changes similar to Word in our interactions with the chat. However, we would not be able to understand the rationale for the changes made, as we would with tools such as Clara or Artext designed by language professionals. One limiting option is to ask the chat to justify each of these changes, but the interaction would become cumbersome, complex and inefficient, not to mention the excessive enthusiasm with which the model would try to justify its corrections.
Examples of generative clarification
Beyond the speed of transformation, generative AI has other advantages over analytics, such as certain elements that can only be identified with GPT capabilities. For example, detecting in a text whether an acronym or acronym has been developed previously, or whether a technicality is explained immediately after its appearance. This requires very complex semantic analysis for analytical AI or rule-based models. In contrast, a great language model is able to establish an intelligent relationship between the acronym and its development, or between the technicality and its meaning, to recognise if this explanation exists somewhere in the text, and to add it where relevant.
Open data to inform clarification
Universal access to open data, especially when it is ready for computational processing, makes it indispensable for training large linguistic models. Huge sources of unstructured information such as Wikipedia, the Common Crawl project or Gutenberg allow systems to learn how the language works. And, on this generalist basis, it is possible to fit models with specialised datasets to make them more accurate in the task of clarifying text.
In the application of generative artificial intelligence to plain language we have the perfect example of a valuable purpose, useful to citizens and positive for social development. Beyond the fascination it has aroused, we have the opportunity to use its potential in a use case that favours equality and inclusiveness. The technology exists, we just need to go down the difficult road of integration.
Content prepared by Carmen Torrijos, expert in AI applied to language and communication.
The contents and points of view reflected in this publication are the sole responsibility of the author.