Using Pandas for quality error reduction in data repositories
Fecha de la noticia: 06-11-2024
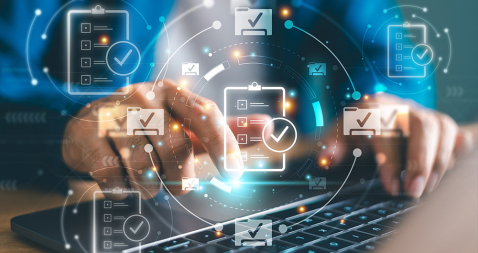
There is no doubt that data has become the strategic asset for organisations. Today, it is essential to ensure that decisions are based on quality data, regardless of the alignment they follow: data analytics, artificial intelligence or reporting. However, ensuring data repositories with high levels of quality is not an easy task, given that in many cases data come from heterogeneous sources where data quality principles have not been taken into account and no context about the domain is available.
To alleviate as far as possible this casuistry, in this article, we will explore one of the most widely used libraries in data analysis: Pandas. Let's check how this Python library can be an effective tool to improve data quality. We will also review the relationship of some of its functions with the data quality dimensions and properties included in the UNE 0081 data quality specification, and some concrete examples of its application in data repositories with the aim of improving data quality.
Using Pandas for data profiling
Si bien el data profiling y la evaluación de calidad de datos están estrechamente relacionados, sus enfoques son diferentes:
- Data Profiling: is the process of exploratory analysis performed to understand the fundamental characteristics of the data, such as its structure, data types, distribution of values, and the presence of missing or duplicate values. The aim is to get a clear picture of what the data looks like, without necessarily making judgements about its quality.
- Data quality assessment: involves the application of predefined rules and standards to determine whether data meets certain quality requirements, such as accuracy, completeness, consistency, credibility or timeliness. In this process, errors are identified and actions to correct them are determined. A useful guide for data quality assessment is the UNE 0081 specification.
It consists of exploring and analysing a dataset to gain a basic understanding of its structure, content and characteristics, before conducting a more in-depth analysis or assessment of the quality of the data. The main objective is to obtain an overview of the data by analysing the distribution, types of data, missing values, relationships between columns and detection of possible anomalies. Pandas has several functions to perform this data profiling.
En resumen, el data profiling es un paso inicial exploratorio que ayuda a preparar el terreno para una evaluación más profunda de la calidad de los datos, proporcionando información esencial para identificar áreas problemáticas y definir las reglas de calidad adecuadas para la evaluación posterior.
What is Pandas and how does it help ensure data quality?
Pandas is one of the most popular Python libraries for data manipulation and analysis. Its ability to handle large volumes of structured information makes it a powerful tool in detecting and correcting errors in data repositories. With Pandas, complex operations can be performed efficiently, from data cleansing to data validation, all of which are essential to maintain quality standards. The following are some examples of how to improve data quality in repositories with Pandas:
1. Detection of missing or inconsistent values: One of the most common data errors is missing or inconsistent values. Pandas allows these values to be easily identified by functions such as isnull() or dropna(). This is key for the completeness property of the records and the data consistency dimension, as missing values in critical fields can distort the results of the analyses.
-
# Identify null values in a dataframe.
df.isnull().sum()
2. Data standardisation and normalisation: Errors in naming or coding consistency are common in large repositories. For example, in a dataset containing product codes, some may be misspelled or may not follow a standard convention. Pandas provides functions like merge() to perform a comparison with a reference database and correct these values. This option is key to maintaining the dimension and semantic consistency property of the data.
# Substitution of incorrect values using a reference table
df = df.merge(product_codes, left_on='product_code', right_on='ref_code', how= 'left')
3. Validation of data requirements: Pandas allows the creation of customised rules to validate the compliance of data with certain standards. For example, if an age field should only contain positive integer values, we can apply a function to identify and correct values that do not comply with this rule. In this way, any business rule of any of the data quality dimensions and properties can be validated.
# Identify records with invalid age values (negative or decimals)
age_errors = df[(df['age'] < 0) | (df['age'] % 1 != 0)])
4. Exploratory analysis to identify anomalous patterns: Functions such as describe() or groupby() in Pandas allow you to explore the general behaviour of your data. This type of analysis is essential for detecting anomalous or out-of-range patterns in any data set, such as unusually high or low values in columns that should follow certain ranges.
# Statistical summary of the data
df.describe()
#Sort by category or property
df.groupby()
5. Duplication removal: Duplicate data is a common problem in data repositories. Pandas provides methods such as drop_duplicates() to identify and remove these records, ensuring that there is no redundancy in the dataset. This capacity would be related to the dimension of completeness and consistency.
# Remove duplicate rows
df = df.drop_duplicates()
Practical example of the application of Pandas
Having presented the above functions that help us to improve the quality of data repositories, we now consider a case to put the process into practice. Suppose we are managing a repository of citizens' data and we want to ensure:
- Age data should not contain invalid values (such as negatives or decimals).
- That nationality codes are standardised.
- That the unique identifiers follow a correct format.
- The place of residence must be consistent.
With Pandas, we could perform the following actions:
1. Age validation without incorrect values:
# Identify records with ages outside the allowed ranges (e.g. less than 0 or non-integers)
age_errors = df[(df['age'] < 0) | (df['age'] % 1 != 0)])
2. Correction of nationality codes:
# Use of an official dataset of nationality codes to correct incorrect entries
df_corregida = df.merge(nacionalidades_ref, left_on='nacionalidad', right_on='codigo_ref', how='left')
3. Validation of unique identifiers:
# Check if the format of the identification number follows a correct pattern
df['valid_id'] = df['identificacion'].str.match(r'^[A-Z0-9]{8}$')
errores_id = df[df['valid_id'] == False]
4. Verification of consistency in place of residence:
# Detect possible inconsistencies in residency (e.g. the same citizen residing in two places at the same time).
duplicados_residencia = df.groupby(['id_ciudadano', 'fecha_residencia'])['lugar_residencia'].nunique()
inconsistencias_residencia = duplicados_residencia[duplicados_residencia > 1]
Integration with a variety of technologies
Pandas is an extremely flexible and versatile library that integrates easily with many technologies and tools in the data ecosystem. Some of the main technologies with which Pandas is integrated or can be used are:
- SQL databases:
Pandas integrates very well with relational databases such as MySQL, PostgreSQL, SQLite, and others that use SQL. The SQLAlchemy library or directly the database-specific libraries (such as psycopg2 for PostgreSQL or sqlite3) allow you to connect Pandas to these databases, perform queries and read/write data between the database and Pandas.
- Common function: pd.read_sql() to read a SQL query into a DataFrame, and to_sql() to export the data from Pandas to a SQL table.
- REST and HTTP-based APIs:
Pandas can be used to process data obtained from APIs using HTTP requests. Libraries such as requests allow you to get data from APIs and then transform that data into Pandas DataFrames for analysis.
- Big Data (Apache Spark):
Pandas can be used in combination with PySpark, an API for Apache Spark in Python. Although Pandas is primarily designed to work with in-memory data, Koalas, a library based on Pandas and Spark, allows you to work with Spark distributed structures using a Pandas-like interface. Tools like Koalas help Pandas users scale their scripts to distributed data environments without having to learn all the PySpark syntax.
- Hadoop and HDFS:
Pandas can be used in conjunction with Hadoop technologies, especially the HDFS distributed file system. Although Pandas is not designed to handle large volumes of distributed data, it can be used in conjunction with libraries such as pyarrow or dask to read or write data to and from HDFS on distributed systems. For example, pyarrow can be used to read or write Parquet files in HDFS.
- Popular file formats:
Pandas is commonly used to read and write data in different file formats, such as:
- CSV: pd.read_csv()
- Excel: pd.read_excel() and to_excel().
- JSON: pd.read_json()
- Parquet: pd.read_parquet() for working with space and time efficient files.
- Feather: a fast file format for interchange between languages such as Python and R (pd.read_feather()).
- Data visualisation tools:
Pandas can be easily integrated with visualisation tools such as Matplotlib, Seaborn, and Plotly.. These libraries allow you to generate graphs directly from Pandas DataFrames.
- Pandas includes its own lightweight integration with Matplotlib to generate fast plots using df.plot().
- For more sophisticated visualisations, it is common to use Pandas together with Seaborn or Plotly for interactive graphics.
- Machine learning libraries:
Pandas is widely used in pre-processing data before applying machine learning models. Some popular libraries with which Pandas integrates are:
- Scikit-learn: la mayoría de los pipelines de machine learning comienzan con la preparación de datos en Pandas antes de pasar los datos a modelos de Scikit-learn.
- TensorFlow y PyTorch: aunque estos frameworks están más orientados al manejo de matrices numéricas (Numpy), Pandas se utiliza frecuentemente para la carga y limpieza de datos antes de entrenar modelos de deep learning.
- XGBoost, LightGBM, CatBoost: Pandas supports these high-performance machine learning libraries, where DataFrames are used as input to train models.
- Jupyter Notebooks:
Pandas is central to interactive data analysis within Jupyter Notebooks, which allow you to run Python code and visualise the results immediately, making it easy to explore data and visualise it in conjunction with other tools.
- Cloud Storage (AWS, GCP, Azure):
Pandas can be used to read and write data directly from cloud storage services such as Amazon S3, Google Cloud Storage and Azure Blob Storage. Additional libraries such as boto3 (for AWS S3) or google-cloud-storage facilitate integration with these services. Below is an example for reading data from Amazon S3.
import pandas as pd
import boto3
#Create an S3 client
s3 = boto3.client('s3')
#Obtain an object from the bucket
obj = s3.get_object(Bucket='mi-bucket', Key='datos.csv')
#Read CSV file from a DataFrame
df = pd.read_csv(obj['Body'])
10. Docker and containers:
Pandas can be used in container environments using Docker.. Containers are widely used to create isolated environments that ensure the replicability of data analysis pipelines .
In conclusion, the use of Pandas is an effective solution to improve data quality in complex and heterogeneous repositories. Through clean-up, normalisation, business rule validation, and exploratory analysis functions, Pandas facilitates the detection and correction of common errors, such as null, duplicate or inconsistent values. In addition, its integration with various technologies, databases, big dataenvironments, and cloud storage, makes Pandas an extremely versatile tool for ensuring data accuracy, consistency and completeness.
Content prepared by Dr. Fernando Gualo, Professor at UCLM and Data Governance and Quality Consultant. The content and point of view reflected in this publication is the sole responsibility of its author.