Artificial intelligence impact on business value chain
Fecha de la noticia: 21-08-2018
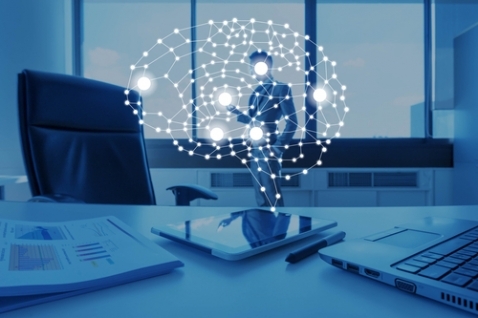
The commercial adoption of any new technology and, therefore, its incorporation into the business value chain follows a cycle that can be moulded in different ways. One of the best known models is the Gartner hype cycle. With regard to artificial intelligence and data science, the current discussion focuses on whether the peak of inflated expectations has already been reached or, on the contrary, we will continue to see how the promises of new and revolutionary innovations increase.
As we advance in this cycle, it is usual to find new advances in technology (new algorithms, in the case of Artificial Intelligence) or a great knowledge about their possibilities of commercial application (new products or products with better price or functionalities). And, of course, the more industries and sectors are affected, the higher expectations are generated.
However, the new discoveries do not only remain on the technological level, but it usually also go deeper into the study and understanding of the economic, social, legal or ethical impact derived from the innovations that are arriving on the market. For any business, it is essential to detect and understand as soon as possible the impact that a new technology will have on its value chain. This way, the company will be able to incorporate the technology into its capabilities before its competitors and generate competitive advantages.
One of the most interesting thesis recently published to model and understand the economic impact of Artificial Intelligence is the one proposed by Professor Ajay Agrawal with Joshua Gans and Avi Goldfarb in his book "Prediction Machines: The Simple Economics of Artificial Intelligence”. The premise is very simple: at the beginning, it establish that the purpose of artificial intelligence, from a merely economic point of view, is to reduce the cost of predictions.
When the cost of a raw material or technology is reduced, it is usual for the industry to increase their use, first applying this technology to the products or services it was designed for, and later, to other product or services that were manufactured in another way. Sometimes it even affects the value of substitute products (that fall) and complementary products (that rise), or other elements of the value chain.
Although these technologies are very complex, the authors were able to establish a surprisingly simple economic framework to understand the AI. But let's see a concrete case, familiar to all of us, in which the increase of the accuracy of the predictions, taken to the extreme, could mean not only to automate a series of tasks, but also to completely change the rules of a business .
As we all know, Amazon uses Artificial Intelligence for the purchase recommendation system that offers suggestions for new products. As mentioned by the authors in his book, the accuracy of this system is around 5%. This means that users acquire 1 out of every 20 products that Amazon suggests, which is not bad.
If Amazon is able to increase the accuracy of these predictions, let's say to 20%, that is, if users acquire 1 out of every 5 suggested products, Amazon would increase its profits enormously and the value of the company would skyrocket even more. But if we imagine a system capable of having a precision of 90% in the purchase predictions, Amazon could consider radically changing its business model and send us products before we decide to buy them, because we would only return 1 out of every 10. AI would not just automate tasks or improve our shopping experience, it would also radically change the way we understand the retail industry.
Given that the main substitute for AI predictions are human predictions, it seems clear that our value as a predictive tool will continue decreasing. The advance of the wave of automations based on artificial intelligence and data science already allows us to see the beginning of this trend.
On the contrary, company data would become an increasingly valuable asset, since they are the main complementary product necessary to generate correct predictions. Likewise, the public data necessary to enrich the companies data, and thus make possible new use cases, would also increase its value.
Following this line of reasoning, we could dare to establish metrics to measure the value of public data where they were used. We would only have to answer this question: how much improves the accuracy of a certain prediction if we enrich the training with determined open data? These improvements would have a concrete value that could give us an idea of the economic value of a public dataset in a specific scenario.
The repeated mantra "data is the new oil" is changing from being a political or marketing affirmation to being supported by economic science, because data are the necessary and indispensable raw material to make good and valuable predictions. And it seems clear that, to continue reducing the predictions cost, the data value should increase. Simple economy.
Content prepared by Jose Luis Marín, Head of Corporate Technology Startegy en MADISON MK and Euroalert CEO.
Contents and points of view expressed in this publication are the exclusive responsibility of its author.