Linguistic corpora: the knowledge engine for AI
Fecha de la noticia: 16-05-2024
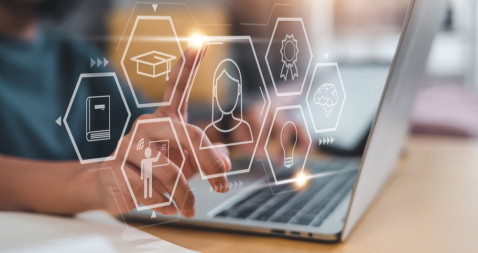
The transfer of human knowledge to machine learning models is the basis of all current artificial intelligence. If we want AI models to be able to solve tasks, we first have to encode and transmit solved tasks to them in a formal language that they can process. We understand as a solved task information encoded in different formats, such as text, image, audio or video. In the case of language processing, and in order to achieve systems with a high linguistic competence so that they can communicate with us in an agile way, we need to transfer to these systems as many human productions in text as possible. We call these data sets the corpus.
Corpus: text datasets
When we talk about corpora (its Latin plural) or datasets that have been used to train Large Language Models (LLMs) such as GPT-4, we are talking about books of all kinds, content written on websites, large repositories of text and information in the world such as Wikipedia, but also less formal linguistic productions such as those we write on social networks, in public reviews of products or services, or even in emails. This variety allows these language models to process and handle text in different languages, registers and styles.
For people working in Natural Language Processing (NLP), data science and data engineering, great enablers like Kaggle or repositories like Awesome Public Datasets on GitHub, which provide direct access to download public datasets. Some of these data files have been prepared for processing and are ready for analysis, while others are in an unstructured state, which requires prior cleaning and sorting before they can be worked with. While also containing quantitative numerical data, many of these sources present textual data that can be used to train language models.
The problem of legitimacy
One of the complications we have encountered in creating these models is that text data that is published on the internet and has been collected via API (direct connections that allow mass downloading from a website or repository) or other techniques, are not always in the public domain. In many cases, they are copyrighted: writers, translators, journalists, content creators, scriptwriters, illustrators, designers and also musicians claim licensing fees from the big tech companies for the use of their text and image content to train models. The media, in particular, are actors greatly impacted by this situation, although their positioning varies according to their situation and different business decisions. There is therefore a need for open corpora that can be used for these training tasks, without prejudice to intellectual property.
Characteristics suitable for a training corpus
Most of the characteristics, which have traditionally have traditionally defined a good corpus in linguistic in linguistic research have not changed when these text datasets are now used to train language models.
- It is still beneficial to use whole texts rather than fragments to ensure coherence.
- Texts must be authentic, from linguistic reality and natural language situations, retrievable and verifiable.
- It is important to ensure a wide diversity in the provenance of texts in terms of sectors of society, publications, local varieties of languages and issuers or speakers.
- In addition to general language, a wide variety of specialised language, technical terms and texts specific to different areas of knowledge should be included.
- Register is fundamental in a language, so we must cover both formal and informal register, in its extremes and intermediate regions.
- Language must be well-formed to avoid interference in learning, so it is desirable to remove code marks, numbers or symbols that correspond to digital metadata and not to the natural formation of the language.
Like specific recommendations for the formats of the files that are to form part of these corpora to be part of these corpora, we find that text corpora with annotations should be stored in UTF-8 encoding and in JSON or CSV format, not in PDF. The preferred format of the sound corpus is WAV 16 bit, 16 KHz. (for voice) or 44.1 KHz (for music and audio). Video corpora should be compiled in MPEG-4 (MP4) format, and translation memories in TMX or CSV.
The text as a collective heritage
National libraries in Europe are actively digitising their rich repositories of history and culture, ensuring public access and preservation. Institutions such as the National Library of France or the British Library are leading the way with initiatives that digitise everything from ancient manuscripts to current web publications. This digital hoarding not only protects heritage from physical deterioration, but also democratises access for researchers and the public and, for some years now, also allows the collection of training corpora for artificial intelligence models.
The corpora provided officially by national libraries allow text collections to be used to create public technology available to all: a collective cultural heritage that generates a new collective heritage, this time a technologicalone. The gain is greatest when these institutional corpora do focus on complying with intellectual property laws, providing only open data and texts free of copyright restrictions, with prescribed or licensed rights. This, coupled with the encouraging fact that the amount of real data needed to train language models is hopefully decreasing as technology advances models is decreasing as technology advances, e.g. with the generation ofadvances, for example, with the generation of synthetic data or the optimisation of certain parameters, indicates that it is possible to train large text models without infringing on intellectual property laws operating in Europe.
In particular, the Biblioteca Nacional de España is making a major digitisation effort to make its valuable text repositories available for research, and in particular for language technologies. Since the first major mass digitisation of physical collections in 2008, the BNE has opened up access to millions of documents with the sole aim of sharing and universalising knowledge. In 2023, thanks to investment from the European Union's Recovery, Transformation and Resilience funds, the BNE is promoting a new digital preservation project in its Strategic Plan 2023-2025the plan focuses on four axes:
- the massive and systematic digitisation of collections,
- BNELab as a catalyst for innovation and data reuse in digital ecosystems,
- partnerships and new cooperation environments,
- and technological integration and sustainability.
The alignment of these four axes with new artificial intelligence and natural language processing technologies is more than obvious, as one of the main data reuses is the training of large language models. Both the digitised bibliographic records and the Library's cataloguing indexes are valuable materials for knowledge technology.
Spanish language models
In 2020, as a pioneering and relatively early initiative, in Spain the following was introduced MarIA a language model promoted by the Secretary of State for Digitalisation and Artificial Intelligence and developed by the National Supercomputing Centre (BSC-CNS), based on the archives of the National Library of Spain. In this case, the corpus was composed of texts from web pages, which had been collected by the BNE since 2009 and which had served to nourish a model originally based on GPT-2.
A lot has happened between the creation of MarIA and the announcement at the announcement at the 2024 Mobile World Congress of the construction of a great foundational language model, specifically trained in Spanish and co-official languages. This system will be open source and transparent, and will only use royalty-free content in its training. This project is a pioneer at European level, as it seeks to provide an open, public and accessible language infrastructure for companies. Like MarIA, the model will be developed at the BSC-CNS, working together with the Biblioteca Nacional de España and other actors such as the Academia Española de la Lengua and the Asociación de Academias de la Lengua Española.
In addition to the institutions that can provide linguistic or bibliographic collections, there are many more institutions in Spain that can provide quality corpora that can also be used for training models in Spanish. The Study on reusable data as a language resource, published in 2019 within the framework of the Language Technologies Plan, already pointed to different sources: the patents and technical reports of the Spanish and European Patent and Trademark Office, the terminology dictionaries of the Terminology Centre, or data as elementary as the census of the National Statistics Institute, or the place names of the National Geographic Institute. When it comes to audiovisual content, which can be transcribed for reuse, we have the video archive of RTVE A la carta, the Audiovisual Archive of the Congress of Deputies or the archives of the different regional television stations. The Boletín Oficial del Estado itself and its associated materials are an important source of textual information containing extensive knowledge about our society and its functioning. Finally, in specific areas such as health or justice, we have the publications of the Spanish Agency of Medicines and Health Products, the jurisprudence texts of the CENDOJ or the recordings of court hearings of the General Council of the Judiciary.
European initiatives
In Europe there does not seem to be as clear a precedent as MarIA or the upcoming GPT-based model in Spanish, as state-driven projects trained with heritage data, coming from national libraries or official bodies.
However, in Europe there is good previous work on the availability of documentation that could now be used to train European-founded AI systems. A good example is the europeana project, which seeks to digitise and make accessible the cultural and artistic heritage of Europe as a whole. It is a collaborative initiative that brings together contributions from thousands of museums, libraries, archives and galleries, providing free access to millions of works of art, photographs, books, music pieces and videos. Europeana has almost 25 million documents in text, which could be the basis for creating multilingual or multilingual competent foundational models in the different European languages.
There are also non-governmental initiatives, but with a global impact, such as Common Corpus which are the ultimate proof that it is possible to train language models with open data and without infringing copyright laws. Common Corpus was released in March 2024, and is the largest dataset created for training large language models, with 500 billion words from various cultural heritage initiatives. This corpus is multilingual and is the largest to date in English, French, Dutch, Spanish, German, Italian and French.
And finally, beyond text, it is possible to find initiatives in other formats such as audio, which can also be used to train AI models. In 2022, the National Library of Sweden provided a sound corpus of more than two million hours of recordings from local public radio, podcasts and audiobooks. The aim of the project was to generate an AI-based model of language-competent audio-to-text transcription that maximises the number of speakers to achieve a diverse and democratic dataset available to all.
Until now, the sense of collectivity and heritage has been sufficient in collecting and making data in text form available to society. With language models, this openness achieves a greater benefit: that of creating and maintaining technology that brings value to people and businesses, fed and enhanced by our own linguistic productions.
Content prepared by Carmen Torrijos, expert in AI applied to language and communication. The contents and points of view reflected in this publication are the sole responsibility of the author.