Peeling the onion of open data governance
Fecha de la noticia: 30-05-2022
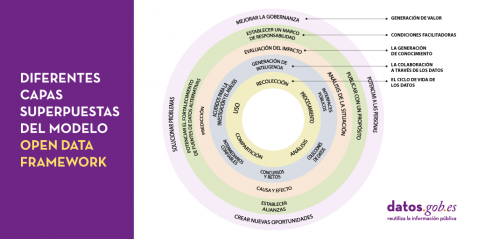
One of the key actions that we recently highlighted as necessary to build the future of open data in our country is the implementation of processes to improve data management and governance. It is no coincidence that proper data management in our organisations is becoming an increasingly complex and in-demand task. Data governance specialists, for example, are increasingly in demand - with more than 45,000 active job openings in the US for a role that was virtually non-existent not so long ago - and dozens of data management platforms now advertise themselves as data governance platforms.
But what's really behind these buzzwords - what is it that we really mean by data governance? In reality, what we are talking about is a series of quite complex transformation processes that affect the whole organisation.
This complexity is perfectly reflected in the framework proposed by the Open Data Policy Lab, where we can clearly see the different overlapping layers of the model and what their main characteristics are - leading to a journey through the elaboration of data, collaboration with data as the main tool, knowledge generation, the establishment of the necessary enabling conditions and the creation of added value.
Let's now peel the onion and take a closer look at what we will find in each of these layers:
The data lifecycle
We should never consider data as isolated elements, but as part of a larger ecosystem, which is embedded in a continuous cycle with the following phases:
- Collection or collation of data from different sources.
- Processing and transformation of data to make it usable.
- Sharing and exchange of data between different members of the organisation.
- Analysis to extract the knowledge being sought.
- Using data according to the knowledge obtained.
Collaboration through data
It is not uncommon for the life cycle of data to take place solely within the organisation where it originates. However, we can increase the value of that data exponentially, simply by exposing it to collaboration with other organisations through a variety of mechanisms, thus adding a new layer of management:
- Public interfaces that provide selective access to data, enabling new uses and functions.
- Trusted intermediaries that function as independent data brokers. These brokers coordinate the use of data by third parties, ensuring its security and integrity at all times.
- Data pooling that provide a common, joint, complete and coherent view of data by aggregating portions from different sources.
- Research and analysis partnership, granting access to certain data for the purpose of generating specific knowledge.
- Prizes and challenges that give access to specific data for a limited period of time to promote new innovative uses of data.
- Intelligence generation, whereby the knowledge acquired by the organisation through the data is also shared and not just the raw material.
Insight generation
Thanks to the collaborations established in the previous layer, it will be possible to carry out new studies of the data that will allow us both to analyse the past and to try to extrapolate the future using various techniques such as:
- Situational analysis, knowing what is happening in the data environment.
- Cause and effect insigths, looking for an explanation of the origin of what is happening.
- Prediction, trying to infer what will happen next.
- Impact assessment, establishing what we expect should happen.
Enabling conditions
There are a number of procedures that when applied on top of an existing collaborative data ecosystem can lead to even more effective use of data through techniques such as:
- Publish with a purpose, with the aim of coordinating data supply and demand as efficiently as possible.
- Foster partnerships, including in our analysis those groups of people and organisations that can help us better understand real needs.
- Prioritize subnational efforts, strengthening of alternative data sources by providing the necessary resources to create new data sources in untapped areas.
- Center data responsability, establishing an accountability framework around data that takes into account the principles of fairness, engagement and transparency.
Value generation
Scaling up the ecosystem -and establishing the right conditions for that ecosystem to flourish- can lead to data economies of scale from which we can derive new benefits such as:
- Improving governance and operations of the organisation itself through the overall improvements in transparency and efficiency that accompany openness processes.
- Empowering people by providing them with the tools they need to perform their tasks in the most appropriate way and make the right decisions.
- Creating new opportunities for innovation, the creation of new business models and evidence-led policy making.
- Solving problems by optimising processes and services and interventions within the system in which we operate.
As we can see, the concept of data governance is actually much broader and more complex than one might initially expect and encompasses a number of key actions and tasks that in most organisations it will be practically impossible to try to centralise in a single role or through a single tool. Therefore, when establishing a data governance system in an organisation, we should face the challenge as an integral transformation process or a paradigm shift in which practically all members of the organisation should be involved to a greater or lesser extent. A good way to face this challenge with greater ease and better guarantees would be through the adoption and implementation of some of the frameworks and reference standards that have been created in this respect and that correspond to different parts of this model.
Content prepared by Carlos Iglesias, Open data Researcher and consultant, World Wide Web Foundation.
The contents and views expressed in this publication are the sole responsibility of the author.