How to start a data analytics project
Fecha de la noticia: 05-09-2018
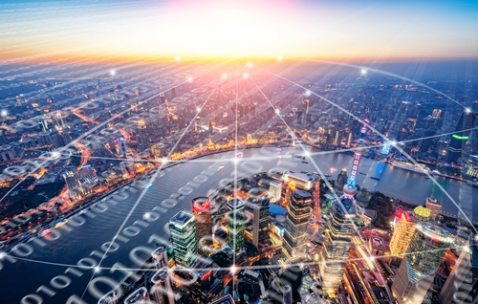
From managing the transport network or sanitary inspections, to predicting the future needs of the personnel. Data analytics can be a powerful tool to optimize municipal policies, guaranteeing a more agile and efficient response to the demands of citizens. More and more municipalities are betting on technologies such as Big Data, artificial intelligence or machine learning in order to improve the governance of the city. However, many times, decision makers do not know how to approach an initiative of this type.
In this context, the Civic Analytics Network has developed the report informe How to Do Data Analytics in Government, a reflection on how data-driven innovations and technology affect municipal government. Through multiple examples, the report recommends us to follow a systematic approach based on 5 steps. These steps can be replicated by any type of initiative, regardless of their level of maturity.
Step 1: Identify the problem
Many analytical projects are based on the desire to give certain data sets a usefulness. However, the project must be approached in another way: the first step is to identify the problem to be solved and, then, identify which are the data sets necessary to solve the problem.
In this phase, data scientists must be involved. They must work together with the different departments to meet the specific needs of users. Likewise, it is important to have the support of high management. Only if all the people is aligned, the problem could be solved.
Step 2: Assess Data Readiness
Next, data scientists will proceed to identify the state of the data sets needed to solve the problem. To do this, the University of Chicago has developed a framework to measure the data maturity and availability. This model uses 3 scorecard matrices: problem definition, data and technology readiness, and organizational readines. Through a series of questions, we can know how data is stored, what information is collected, privacy and documentation practices, what personnel participate in each task, data use policy, as well as who leads the project or who finances it.
Step 3: Scope the project
In this phase, we will determinate the necessary actions to achieve our goal. If in the previous phase we have determined what data we have and how they are, in this phase we will have to determine if those data are enough or if it is necessary to use any additional source. Likewise, we also address the type of analysis to be done (descriptive, predictive or prescriptive) or how the analysis will be validated.
Step 4: Pilot the project
This phase could also be called "the trial and error phase". Implementing the project on a small scale and in a real environment, we can see how it behaves and solve the problems that may arise. As good as we have defined the scope of the project, the pilot may not work as expected, either because an assumption is incorrect or because an algorithm error.
Step 5: Implement and Scale the Model
Once the pilot starts giving results it is time to scale the project to other areas, replicating the model.
The report also shows multiple examples of analytical projects that have helped optimize public policies. For example, New Orleans has developed a model to determine the distribution of smoke detectors, while Chicago uses predictive analysis to prepare for a peak of patients affected by a particular virus. Many of these initiatives could be replicated in our country, where we already found some examples such as Predict, a tool that predict pollution levels to facilitate traffic management.
The continuous adoption of analysis solutions in the governments of the cities does not show signs of deceleration, but quite the opposite. To be successful in these projects, it is necessary to approach them in a structured way, dedicating their time to each phase and involving all the necessary roles.