Data documentation: Datasheets for datasets
Fecha de la noticia: 06-10-2022
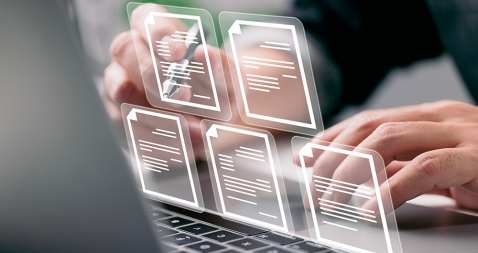
16.5 billion euros. These are the revenues that artificial intelligence (AI) and data are expected to generate in Spanish industry by 2025, according to what was announced last February at the IndesIA forum, the association for the application of artificial intelligence in industry. AI is already part of our daily lives: either by making our work easier by performing routine and repetitive tasks, or by complementing human capabilities in various fields through machine learning models that facilitate, for example, image recognition, machine translation or the prediction of medical diagnoses. All of these activities help us to improve the efficiency of businesses and services, driving more accurate decision-making.
But for machine learning models to work properly, they need quality and well-documented data. Every machine learning model is trained and evaluated with data. The characteristics of these datasets condition the behaviour of the model. For example, if the training data reflects unwanted social biases, these are likely to be incorporated into the model as well, which can have serious consequences when used in high-profile areas such as criminal justice, recruitment or credit lending. Moreover, if we do not know the context of the data, our model may not work properly, as its construction process has not taken into account the intrinsic characteristics of the data on which it is based.
For these and other reasons, the World Economic Forum suggests that all entities should document the provenance, creation and use of machine learning datasets in order to avoid erroneous or discriminatory results..
What are datasheets for datasets?
One mechanism for documenting this information is known as Datasheets for datasets. This framework proposes that every dataset should be accompanied by a datasheet, which consists of a questionnaire that guides data documentation and reflection throughout the data lifecycle. Some of the benefits are:
- It improves collaboration, transparency and accountability within the machine learning community.
- Mitigates unwanted social biases in models.
- Helps researchers and developers select the most appropriate datasets to achieve their specific goals.
- Facilitates greater reproducibility of results.
Datasheets will vary depending on factors such as knowledge area, existing organisational infrastructure or workflows.
To assist in the creation of datasheets, a questionnaire has been designed with a series of questions, according to the stages of the data lifecycle:
- Motivation. Collects the reasons that led to the creation of the datasets. It also asks who created or funded the datasets.
- Composition. Provides users with the necessary information on the suitability of the dataset for their purposes. It includes, among other questions, which units of observation represent the dataset (documents, photos, persons, countries), what kind of information each unit provides or whether there are errors, sources of noise or redundancies in the dataset. Reflect on data that refer to individuals to avoid possible social biases or privacy violations.
- Collection process. It is intended to help researchers and users think about how to create alternative datasets with similar characteristics. It details, for example, how the data were acquired, who was involved in the collection process, or what the ethical review process was like. It deals especially with the ethical aspects of processing data protected by the GDPR.
- Preprocessing, cleansing or tagging. These questions allow data users to determine whether data have been processed in ways that are compatible with their intended uses. Inquire whether any preprocessing, cleansing or tagging of the data was performed, or whether the software that was used to preprocess, cleanse and tag the data is available.
- Uses. This section provides information on those tasks for which the data may or may not be used. For this, questions such as: Has the dataset already been used for any task? What other tasks can it be used for? Does the composition of the dataset or the way it was collected, preprocessed, cleaned and labelled affect other future uses?
- Distribution. This covers how the dataset will be disseminated. Questions focus on whether the data will be distributed to third parties and, if so, how, when, what are the restrictions on use and under what licences.
- Maintenance. The questionnaire ends with questions aimed at planning the maintenance of the data and communicating the plan to the users of the data. For example, answers are given to whether the dataset will be updated or who will provide support.
It is recommended that all questions are considered prior to data collection, so that data creators can be aware of potential problems. To illustrate how each of these questions could be answered in practice, the model developers have produced an appendix with an example for a given dataset.
Is Datasheets for datasets effective?
The Datasheets for datasets data documentation framework has initially received good reviews, but its implementation continues to face challenges, especially when working with dynamic data.
To find out whether the framework effectively addresses the documentation needs of data creators and users, in June 2022, Microsoft USA and the University of Michigan conducted a study on its implementation. To do so, they conducted a series of interviews and a follow-up on the implementation of the questionnaire by a number of machine learning professionals.
In summary, participants expressed the need for documentation frameworks to be adaptable to different contexts, to be integrated into existing tools and workflows, and to be as automated as possible, partly due to the length of the questions. However, they also highlighted its advantages, such as reducing the risk of information loss, promoting collaboration between all those involved in the data lifecycle, facilitating data discovery and fostering critical thinking, among others.
In short, this is a good starting point, but it will have to evolve, especially to adapt to the needs of dynamic data and documentation flows applied in different contexts.
Content prepared by the datos.gob.es team.