Data Mesh and Data Fabric: New Perspectives in Enterprise Data Architectures
Fecha de la noticia: 10-01-2025
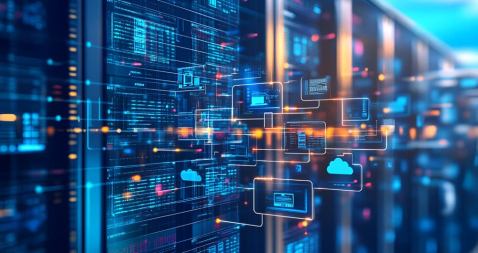
Over the last decade, the amount of data that organisations generate and need to manage has grown exponentially. With the rise of the cloud, Internet of Things (IoT), edge computing and artificial intelligence (AI), enterprises face the challenge of integrating and governing data from multiple sources and environments. In this context, two key approaches to data management have emerged that seek to solve the problems associated with data centralisation: Data Mesh y Data Fabric. Although these concepts complement each other, each offers a different approach to solving the data challenges of modern organisations.
Why is a data lake not enough?
Many companies have implemented data lakes or centralised data warehouses with dedicated teams as a strategy to drive company data analytics. However, this approach often creates problems as the company scales up, for example:
- Centralised data equipment becomes a bottleneck. These teams cannot respond quickly enough to the variety and volume of questions that arise from different areas of the business.
- Centralisation creates a dependency that limits the autonomy of domain teams, who know their data needs best.
This is where the Data Meshapproach comes in.
Data Mesh: a decentralised, domain-driven approach
Data Mesh breaks the centralisation of data and distributes it across specific domains, allowing each business team (or domain team) to manage and control the data it knows and uses most. This approach is based on four basic principles:
- Domain ownership: instead of a central data computer having all the control, each computer is responsible for the data it generates. That is, if you are the sales team, you manage the sales data; if you are the marketing team, you manage the marketing data. Nobody knows this data better than the team that uses it on a daily basis.
- Data as a product: this idea reminds us that data is not only for the use of the domain that generates it, but can be useful for the entire enterprise. So each team should think of its data as a "product" that other teams can also use. This implies that the data must be accessible, reliable and easy to find, almost as if it were a public API.
- Self-service platform: decentralisation does not mean that every team has to reinvent the wheel. To prevent each domain team from specialising in complex data tools, the Data Mesh is supported by a self-service infrastructure that facilitates the creation, deployment and maintenance of data products. This platform should allow domain teams to consume and generate data without relying on high technical expertise.
- Federated governance: although data is distributed, there are still common rules for all. In a Data Mesh, governance is "federated", i.e. each device follows globally defined interoperability standards. This ensures that all data is secure, high quality and compliant.
These principles make Data Mesh an ideal architecture for organisations seeking greater agility and team autonomy without losing sight of quality and compliance. Despite decentralisation, Data Mesh does not create data silos because it encourages collaboration and standardised data sharing between teams, ensuring common access and governance across the organisation.
Data Fabric: architecture for secure and efficient access to distributed data
While the Data Mesh focuses on organising and owning data around domains, the Data Fabric is an architecture that allows connecting and exposing an organisation's data, regardless of its location. Unlike approaches based on data centralisation, such as the data lake, the Data Fabric acts as a unified layer, providing seamless access to data distributed across multiple systems without the need to physically move it to a single repository.
In general terms, the Data Fabric is based on three fundamental aspects:
- Access to data: in a modern enterprise, data is scattered across multiple locations, such as data lakes, data warehouses, relational databases and numerous SaaS (Software as-a-Service) applications. Instead of consolidating all this data in one place, the Data Fabric employs a virtualisation layer that allows it to be accessed directly from its original sources. This approach minimises data duplication and enables real-time access, thus facilitating agile decision-making. In cases where an application requires low latencies, the Data Fabric also has robust integration tools, such as ETL (extract, transform and load), to move and transform data when necessary.
- Data lifecycle management: the Data Fabric not only facilitates access, but also ensures proper management throughout the entire data lifecycle. This includes critical aspects such as governance, privacy and compliance. The architecture of the Data Fabric relies on active metadata that automates the application of security and access policies, ensuring that only users with the appropriate permissions access the corresponding information. It also offers advanced traceability (lineage) functionalities, which allow tracking the origin of data, knowing its transformations and assessing its quality, which is essential in environments regulated under regulations such as the General Data Protection Regulation (GDPR).
- Data exposure: after connecting the data and applying the governance and security policies, the next step of the Data Fabric is to make that data available to end users. Through enterprise catalogues, data is organised and presented in a way that is accessible to analysts, data scientists and developers, who can locate and use it efficiently.
In short, the Data Fabric does not replace data lakes or data warehouses, but facilitates the integration and management of the organisation's existing data. It aims to create a secure and flexible environment that enables the controlled flow of data and a unified view, without the need to physically move it, thus driving more agile and informed decision-making.
Data Mesh vs. Data Fabric. Competitors or allies?
While Data Mesh and Data Fabric have some objectives in common, each solves different problems and, in fact, benefits can be found in applying mechanisms from both approaches in a complementary manner. The following table shows a comparison of the two approaches:
APEARANCE |
DATA MESH |
DATA FABRIC |
Approach |
Organisational and structural, domain-oriented. |
Technical, focusing on data integration. |
Purpose |
Decentralise ownership and responsibility for data to domain teams. |
Create a unified data access layer distributed across multiple environments. |
Data management |
Each domain manages its own data and defines quality standards. |
Data is integrated through services and APIs, allowing a unified view without physically moving data. |
Governance |
Federated, with rules established by each domain, maintaining common standards. |
Centralised at platform level, with automation of access and security policies through active metadata. |
Figure 1. Comparative table of Data Mesh VS. Data Fabric. Source: Own elaboration.
Conclusion
Both Data Mesh and Data Fabric are designed to solve the challenges of data management in modern enterprises. Data Mesh brings an organisational approach that empowers domain teams, while Data Fabric enables flexible and accessible integration of distributed data without the need to physically move it. The choice between the two, or a combination of the two, will depend on the specific needs of each organisation, although it is important to consider the investment in infrastructure, training and possible organisational changes that these approaches require. For small to medium-sized companies, a traditional data warehouse can be a practical and cost-effective alternative, especially if their data volumes and organisational complexity are manageable. However, given the growth of data ecosystems in organisations, both models represent a move towards a more agile, secure and useful data environment, facilitating data management that is better aligned with strategic objectives in an environment.
Definitions
- Data Lake: it is a storage repository that allows large volumes of data to be stored in their original format, whether structured, semi-structured or unstructured. Its flexible structure allows raw data to be stored and used for advanced analytics and machine learning.
- Data Warehouse: it is a structured data storage system that organises, processes and optimises data for analysis and reporting. It is designed for quick queries and analysis of historical data, following a predefined scheme for easy access to information.
References
- Dehghani, Zhamak. Data Mesh Principles and Logical Architecture. https://martinfowler.com/articles/data-mesh-principles.html.
- Dehghani, Zhamak. Data Mesh: Delivering Data-Driven Value at Scale. O'Reilly Media. Book detailing the implementation and fundamental principles of Data Mesh in organisations.
- Data Mesh Architecture. Website about Data Mesh and data architectures. https://www.datamesh-architecture.com/
- IBM Data Fabric. IBM Topics. https://www.ibm.com/topics/data-fabric
- IBM Technology. Data Fabric. Unifying Data Across Hybrid and Multicloud Environments. YouTube. https://www.youtube.com/watch?v=0Zzn4eVbqfk&t=4s&ab_channel=IBMTechnology
Content prepared by Juan Benavente, senior industrial engineer and expert in technologies linked to the data economy. The contents and points of view reflected in this publication are the sole responsibility of the author.