Common mistakes in the development of a data strategy
Fecha del post: 26-12-2024
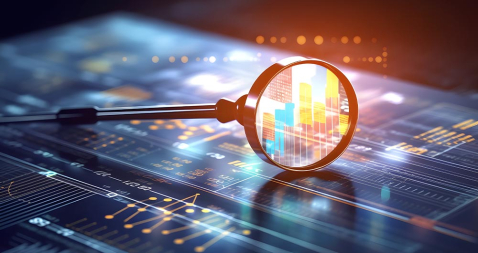
In an increasingly data-driven world, all organisations, both private companies and public bodies, are looking to leverage their information to make better decisions, improve the efficiency of their processes and meet their strategic objectives. However, creating an effective data strategy is a challenge that should not be underestimated.
Often, organisations in all sectors fall into common mistakes that can compromise the success of their strategies from the outset. From ignoring the importance of data governance to not aligning strategic objectives with the real needs of the institution, these failures can result in inefficiencies, non-compliance with regulations and even loss of trust by citizens, employees or users.
In this article, we will explore the most common mistakes in creating a data strategy, with the aim of helping both public and private entities to avoid them. Our goal is to provide you with the tools to build a solid foundation to maximise the value of data for the benefit of your mission and objectives.
Figure 1. Tips for designing a data governance strategy. Source: own elaboration.
The following are some of the most common mistakes in developing a data strategy, justifying their impact and the extent to which they can affect an organisation:
Lack of linkage to organisational objectives and failure to identify key areas
For data strategy to be effective in any type of organisation, it is essential that it is aligned with its strategic objectives. These objectives include key areas such as revenue growth, service improvement, cost optimisation and customer/citizen experience. In addition, prioritising initiatives is essential to identify the areas of the organisation that will benefit most from the data strategy. This approach not only allows maximising the return on data investment, but also ensures that initiatives are clearly connected to desired outcomes, reducing potential gaps between data efforts and strategic objectives.
Failure to define clear short- and medium-term objectives
Defining specific and achievable goals in the early stages of a data strategy is very important to set a clear direction and demonstrate its value from the outset. This boosts the motivation of the teams involved and builds trust between leaders and stakeholders. Prioritising short-term objectives, such as implementing a dashboard of key indicators or improving the quality of a specific set of critical data, delivers tangible results quickly and justifies the investment in the data strategy. These initial achievements not only consolidate management support, but also strengthen the commitment of the teams.
Similarly, medium-term objectives are essential to build on initial progress and prepare the ground for more ambitious projects. For example, the automation of reporting processes or the implementation of predictive models for key areas can be intermediate goals that demonstrate the positive impact of the strategy on the organisation. These achievements allow us to measure progress, evaluate the success of the strategy and ensure that it is aligned with the organisation's strategic priorities.
Setting a combination of short- and medium-term goals ensures that the data strategy remains relevant over time and continues to generate value. This approach helps the organisation to move forward in a structured way, strengthening its position both vis-à-vis its competitors and in fulfilling its mission in the case of public bodies.
Failure to conduct a maturity assessment beforehand to define the strategy as narrowly as possible.
Before designing a data strategy, it is crucial to conduct a pre-assessment to understand the current state of the organisation in terms of data and to realistically and effectively scope it. This step not only prevents efforts from being dispersed, but also ensures that the strategy is aligned with the real needs of the organisation, thus maximising its impact. Without prior assessment, it is easy to fall into the error of taking on initiatives that are too broad or poorly connected to strategic priorities .
Therefore, conducting this pre-assessment is not only a technical exercise, but a strategic tool to ensure that resources and efforts are well targeted from the outset. With a clear diagnosis, the data strategy becomes a solid roadmap, capable of generating tangible results from the earliest stages. It should be recalled that the UNE 0080:2023, which focuses on the assessment of the maturity of data governance and management, provides a structured framework for this initial assessment. This standard allows for an objective analysis of the organisation's processes, technologies and capabilities around data..
Failure to carry out data governance initiatives
The definition of a sound strategy is fundamental to the success of data governance initiatives. It is essential to have an area or unit responsible for data governance, such as a data office or a centre of excellence, where clear guidelines are established and the necessary actions are coordinated to achieve the committed strategic objectives. These initiatives must be aligned with the organisation's priorities, ensuring that the data is secure, usable for its intended purpose and compliant with applicable laws and regulations.
A robust data governance framework is key to ensuring consistency and quality of data, strengthening confidence in reporting and analysis that generates both internal and external value. In addition, an appropriate approach reduces risks such as non-compliance, promoting effective use of data and protecting the organisation's reputation.
It is therefore important to design these initiatives with a holistic approach, prioritising collaboration between different areas and aligning them with the overall data strategy. For more information on how to structure an effective data governance system, see this series of articles: From data strategy to data governance system - Part 1.
Focusing exclusively on technology
Many organisations have the mistaken view that acquiring sophisticated tools and platforms will be the ultimate solution to their data problems. However, technology is only one part of the ecosystem. Without the right processes, governance framework and, of course, people, even the best technology will fail. This is problematic because it can lead to huge investments with no clear return, as well as frustration among teams when they do not get the expected results.
Failure to involve all stakeholders and define roles and responsibilities
A sound data strategy needs to bring together all relevant actors, whether in a public administration or in a private company. Each area, department or unit has a unique vision of how data can be useful to achieve objectives, improve services or make more informed decisions. Therefore, involving all stakeholders from the outset not only enriches the strategy, but also ensures that they are aligned with the real needs of the organisation.
Likewise, defining clear roles and responsibilities is key to avoid confusion and duplication. Knowing who is responsible for the data, who manages it and who uses it ensures a more efficient workflow and fosters collaboration between teams. In both the public and private spheres, this approach helps to maximise the impact of the data strategy, ensuring that efforts are coordinated and focused towards a common goal.
Failure to establish clear metrics of success
Establishing key performance indicators (KPIs) is essential to assess whether initiatives are generating value. KPIs help demonstrate the results of the data strategy, reinforcing leadership support and encouraging willingness to invest in the future. By measuring the impact of actions, organisations can guarantee the sustainability and continuous development of their strategy, ensuring that it is aligned with strategic objectives and delivers tangible benefits.
Failure to place data quality at the centre
A sound data strategy must be built on a foundation of reliable and high quality data. Ignoring this aspect can lead to wrong decisions, inefficient processes and loss of trust in data by teams. Data quality is not just a technical aspect, but a strategic enabler: it ensures that the information used is complete, consistent, valid and timely.
Integrating data quality from the outset involves defining clear metrics, establishing validation and cleansing processes, and assigning responsibilities for their maintenance. Furthermore, by placing data quality at the heart of the strategy, organisations can unlock the true potential of data, ensuring that it accurately supports business objectives and reinforces user confidence. Without quality, the strategy loses momentum and becomes a wasted opportunity.
Failure to manage cultural change and resistance to change
The transition to a data-driven organisation requires not only tools and processes, but also a clear focus on change management to engage employees. Promoting an open mind towards new practices is key to ensuring the adoption and success of the strategy. By prioritising communication, training and team engagement, organisations can facilitate this cultural change, ensuring that all levels work in alignment with strategic objectives and maximising the impact of the data strategy.
Not planning for scalability
It is critical for organisations to consider how their data strategy can scale as the volume of information grows. Designing a strategy ready to handle this growth ensures that systems can support the increase in data without the need for future restructuring, optimising resources and avoiding additional costs. By planning for scalability, organisations can ensure long-term sustainable operational efficiency and maximise the value of their data as their needs evolve.
Lack of continuous updating and review of the strategy
Data and organisational needs are constantly evolving, so it is important to regularly review and adapt the strategy to keep it relevant and effective. A flexible and up-to-date data strategy allows you to respond nimbly to new opportunities and challenges, ensuring that you continue to deliver value as market or organisational priorities change. This proactive approach ensures that the strategy remains aligned with strategic objectives and reinforces its long-term positive impact.
In conclusion, it is important to highlight that the success of a data strategy lies in its ability to align with the strategic objectives of the organisation, setting clear goals and encouraging the participation of all areas involved. A good data governance system, accompanied by metrics to measure its impact, is the basis for ensuring that the strategy generates value and is sustainable over time.
In addition, addressing issues such as data quality, cultural change and scalability from the outset is essential to maximise its effectiveness. Focusing exclusively on technology or neglecting these elements can limit results and jeopardise the organisation's ability to adapt to new opportunities and challenges. Finally, continuously reviewing and updating the strategy ensures its relevance and reinforces its positive impact.
To learn more about how to structure an effective data strategy and its connection with a solid data governance system, we recommend exploring the articles published in datos.gob.es: From Data Strategy to Data Governance System - Part 1 and Part 2.. These resources complement the concepts presented in this article and offer practical insights for implementation in any type of organisation.
Content elaborated by Dr. Fernando Gualo, Professor at UCLM and Data Governance and Quality Consultant. The content and the point of view reflected in this publication are the sole responsibility of its author.