From data strategy to data governance system (part 2)
Fecha de la noticia: 30-10-2023
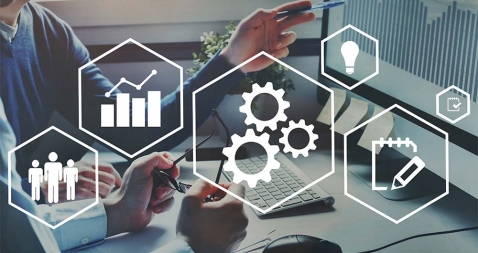
In the first part of this article, the concept of data strategy was introduced as the organisation's effort to put the necessary data at the service of its business strategy. In this second part, we will explore some aspects related to the materialisation of such a strategy as part of the design or maintenance - if it already exists - of a data governance system.
For the materialisation of the data strategy, a development environment will have to be addressed, as described in a founding act that includes some aspects such as the identification of the main people responsible for the implementation, the expected results, the available resources and the timeframe established to achieve the objectives. In addition, it will contain a portfolio of data governance programmes including individual projects or specific related projects to address the achievement of the strategic objectives of the data.
It is important to mention that the implementation of a data strategy has an impact on the development and maintenance of the different components of a data governance system:
- Manager
- Organisational structures
- Principles, policies and frameworks
- For Information
- Culture, ethics and behaviour
- People, skills and competences
- Services, infrastructures and applications.
In this sense, it can be said that each of the projects included in the data government's programme aims to contribute to developing or maintaining one or more of these components.
It should be noted that the final design of this data governance system is achieved in an iterative and incremental manner over time, depending on the constraints and possibilities of the organisation and its current operating context. Consequently, the prioritisation, selection and sequencing of projects within the data governance programme to implement the strategic objectives of data also has an iterative and incremental nature[1].
The three biggest data risks commonly encountered in organisations are:
- Not knowing who has to take responsibility for implementing the data strategy,
- Not having adequate knowledge of data in quantity and quality, and
- Failure to exercise adequate control over the data, e.g. by at least complying with current legislation.
Therefore, as far as possible, projects should be approached in the following way:
- Firstly, to address those projects related to the identification, selection or maintenance of organisational structures ("strategic alignment" type objective), also known as governance framework.
- Next, undertake projects related to the knowledge of the business processes and the data used (a "strategic alignment" type objective aimed at the description of data through the corresponding metadata, including data lifecycle metadata).
- And finally, proceed to the definition of policies and derived controls for different policy areas (which may be of the "strategic alignment", "risk optimisation" or "value for money" type).
The artefact-based approach and the process approach
In approaching the definition of these data governance programmes, some organisations with a project understanding more oriented to the generation and deployment of technological products follow an artefact-based approach. That is, they approach the projects that are part of the data governance programme as the achievement of certain artefacts. Thus, it is possible to find organisations whose first concern when implementing data governance is to acquire and install a specific tool that supports, for example, a glossary of terms, a data dictionary, or a data lake. Moreover, as for various reasons some companies do not adequately differentiate between data governance and data management, this approach is often sufficient. However, the artefact approach introduces the risk of "the tool without the instruction manual the artefact approach introduces the risk of "the tool without the instruction manual": the tool is purchased - probably after a proof of concept by the vendor - and deployed according to the needs of the organisation, but what it is used for and when it is used is unknown, leaving the artefact often as an isolated resource. This, unless the organisation promotes a profound change, may end up being a waste of resources in the long run as the use of the artefacts generated is abandoned.
A better alternative, as has been widely demonstrated in the software development sector, is the execution of the data governance programme with a process approach. This process approach allows not only to develop the necessary artefacts, but also to model the way the organisation works with respect to some area of performance, and contextualises the rationale and use of the artefacts within the process, specifying who should use the artefact, for what, when, what should be obtained by using the artefact, etc.
This process approach is an ideal instrument to capture and model the knowledge that the organisation already has regarding the tasks covered by the process, and to make this knowledge the reference for new operations to be carried out in the future. In addition, the definition of the process also allows for the particularisation of chains of responsibility and accountability and the establishment of communication plans, so that each worker knows what to do, what artefacts to use, who to ask for or receive resources from to carry out their work, who to communicate their results to, or who to escalate potential problems to.
This way of working provides some advantages, such as predictable behaviour of the organisation with respect to the process; the possibility to use these processes as building blocks for the execution of data projects; the option to easily replace a human resource; or the possibility to efficiently measure the performance of a process. But undoubtedly, one of the greatest advantages of this process approach is that it allows organisations to adopt the good practices contained in any of the process reference models for data governance, data management and quality management that exist in the current panorama, such as the UNE 0077 specifications (for data governance), UNE 0078 (for data management) and UNE 0079 (for data quality management).
This adoption enables the possibility of using frameworks for process assessment and improvement, such as the one described in UNE 0080, which includes the Alarcos Data Maturity Model, in which the concept of organisational maturity of data governance, data management and data quality management is introduced as an indicator of the organisation's potential to face the achievement of strategic objectives with certain guarantees of success. In fact, it is common for many organisations adopting the process approach to pre-include specific data objectives ("strategic alignment" type objectives) aimed at preparing the organisation - by increasing the level of maturity - to better support the execution of the data governance programme. These "preparatory" objectives are mainly manifested in the implementation of data governance, data management and data quality management processes to close the gap between the current initial state of maturity (AS_IS) and the required final state of maturity of the organisation (TO_BE).
If the process approach is chosen, the different projects contained in the data programme will generate controlled and targeted increments in each of the components of the data governance system, which will enable the transformation of the organisation to meet the organisation's strategic business objectives.
Ultimately, the implementation of a data strategy manifests itself in the development or maintenance of a data governance system, which ensures that the organisation's data is put at the service of strategic business objectives. The instrument to achieve this objective is the data governance programme, which should ideally be implemented through a process approach in order to benefit from all the advantages it brings.
______________________________________________
Content developed by Dr. Ismael Caballero, Associate Professor at UCLM, and Dr. Fernando Gualo, PhD in Computer Science, and Chief Executive Officer and Data Quality and Data Governance Consultant.
The content and viewpoints reflected in this publication are the sole responsibility of the authors.
[1] We recommend reading the article https://hdl.handle.net/11705/JISBD/2019/083