The importance of data fairness in artificial intelligence systems
Fecha de la noticia: 16-07-2024
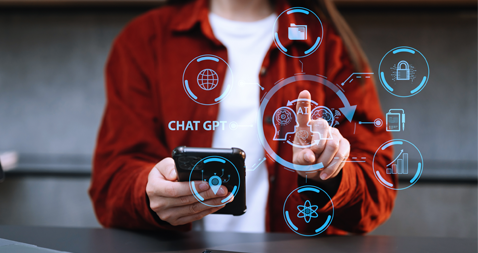
Data equity is a concept that emphasises the importance of considering issues of power, bias and discrimination in data collection, analysis and interpretation. It involves ensuring that data is collected, analysed and used in a way that is fair, inclusive and equitable to all stakeholders, particularly those who have historically been marginalised or excluded. Although there is no consensus on its definition, data equity aims to address systemic inequalities and power imbalances by promoting transparency, accountability and community ownership of data. It also involves recognising and redressing legacies of discrimination through data and ensuring that data is used to support the well-being and empowerment of all individuals and communities. Data equity is therefore a key principle in data governance, related to impacts on individuals, groups and ecosystems
To shed more light on this issue, the World Economic Forum - an organisation that brings together business leaders and experts to organisation that brings together leaders of major companies and experts to discuss global issues - published a short report entitled published a few months ago a short report entitled Data Equity: Foundational Concepts for Generative AI, aimed at industry, civil society, academia and decision-makers.
The aim of the World Economic Forum paper is, first, to define data equity and demonstrate its importance in the development and implementation of Generative AI (known as genAI). In this report, the World Economic Forum identifies some challenges and risks associated with data inequity in AI development, such as bias, discrimination and unfair outcomes. It also aims to provide practical guidance and recommendations for achieving data equity, including strategies for data collection, analysis and use. On the other hand, the World Economic Forum says it wants, on the one hand, to foster collaboration between stakeholders from industry, governments, academia and civil society to address data equity issues and promote the development of fair and inclusive AI, and on the other hand, to influence the future of AI development.
Some of the key findings of the report are discussed below.
Types of data equity
The paper identifies four main classes of data equity:
- Fairness of representation refers to the fair and proportional inclusion of different groups in the datasets used to train genAI models.
- Resource equity refers to the equitable distribution of resources (data, infrastructure and knowledge) necessary for the development and use of genAI.
- Equity of access means ensuring fair and non-discriminatory access to the capabilities and benefits of genAI by different groups.
- Equity of results seeks to ensure that genAI results and applications do not generate disproportionate or detrimental impacts on vulnerable groups.
Equity challenges in the genAI
The paper highlights that foundation models, which are the basis of many genAI tools, present specific data fairness challenges, as they encode biases and prejudices present in training datasets and can amplify them in their results. In AI, a function model refers to a program or algorithm that relies on training data to recognise patterns and make predictions or decisions, allowing it to make predictions or decisions based on new input data.
The main challenges in terms of social justice with artificial intelligence (AI) include thefact thattraining data may be biased. Generative AI models are trained on large datasets that often contain bias and discriminatory content, which can lead to the perpetuation of hate speech, misogyny and racism. Algorithmic biasescan then occur, which not only reproduce these initial biases, but can amplify them, increasing existing social inequalities and resulting in discrimination and unfair treatment of stereotyped groups. There are also privacy concerns, as generative AI relies on some sensitive personal data, which can be exploited and exposed.
The increasing use of generative AI in various fields is already causing job changes, as it is easier, quicker or cheaper to ask an artificial intelligence to create an image or text - in fact, based on human creations that exist on the internet - than to commission an expert to do so. This can exacerbate economic inequalities.
Finally, generative AI has the potential to intensify disinformation. Generative AI can be used to create high-quality deepfakes, which are already being used to spread hoaxes and misinformation, potentially undermining democratic processes and institutions.
Gaps and possible solutions
These challenges highlight the need for careful consideration and regulation of generative AI to ensure that it is developed and used in a way that respects human rights and promotes social justice. However, the document does not address misinformation and only mentions gender when talking about "feature equity", a component of data equity. Equity of characteristics seeks to "ensure accurate representation of the individuals, groups and communities represented by the data, which requires the inclusion of attributes such as race, gender, location and income along with other data" (p.4). Without these attributes, the paper says, "it is often difficult to identify and address latent biases and inequalities". However, the same characteristics can be used to discriminate against women, for example.
Addressing these challenges requires the engagement and collaboration of various stakeholders, such as industry, government, academia and civil society, to develop methods and processes that integrate data equity considerations into all phases of genAIdevelopment. This document lays the theoretical foundations of what can be understood as data equity; however, there is still a long way to go to see how to move from theory to practice in regulation, habits and knowledge.
This document links up with the steps already being taken in Europe and Spain with the European Union's AI Law y the IA Strategy of the Spanish Government respectively. Precisely, one of the axes of the latter (Axis 3) is to promote transparent, ethical and humanistic AI.
The Spanish AI strategy is a more comprehensive document than that of the World Economic Forum, outlining the government's plans for the development and adoption of general artificial intelligence technologies. The strategy focuses on areas such as talent development, research and innovation, regulatory frameworks and the adoption of AI in the public and private sectors, and targets primarily national stakeholders such as government agencies, businesses and research institutions. While the Spanish AI strategy does not explicitly mention data equity, it does emphasise the importance of responsible and ethical AI development, which could include data equity considerations.
The World Economic Forum report can be found here: Data Equity: Foundational Concepts for Generative AI | World Economic Forum (weforum.org)
Content prepared by Miren Gutiérrez, PhD and researcher at the University of Deusto, expert in data activism, data justice, data literacy and gender disinformation. The contents and views reflected in this publication are the sole responsibility of its author.