Open data and generative AI: synergies and use cases
Fecha de la noticia: 04-09-2024
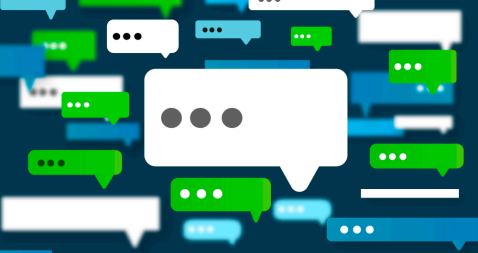
Artificial intelligence (AI) is revolutionising the way we create and consume content. From automating repetitive tasks to personalising experiences, AI offers tools that are changing the landscape of marketing, communication and creativity.
These artificial intelligences need to be trained with data that are fit for purpose and not copyrighted. Open data is therefore emerging as a very useful tool for the future of AI.
The Govlab has published the report "A Fourth Wave of Open Data? Exploring the Spectrum of Scenarios for Open Data and Generative AI" to explore this issue in more detail. It analyses the emerging relationship between open data and generative AI, presenting various scenarios and recommendations. Their key points are set out below.
The role of data in generative AI
Data is the fundamental basis for generative artificial intelligence models. Building and training such models requires a large volume of data, the scale and variety of which is conditioned by the objectives and use cases of the model.
The following graphic explains how data functions as a key input and output of a generative AI system. Data is collected from various sources, including open data portals, in order to train a general-purpose AI model. This model will then be adapted to perform specific functions and different types of analysis, which in turn generate new data that can be used to further train models.
Figure 1. The role of open data in generative AI, adapted from the report “A Fourth Wave of Open Data? Exploring the Spectrum of Scenarios for Open Data and Generative AI”, The Govlab, 2024.
5 scenarios where open data and artificial intelligence converge
In order to help open data providers 'prepare' their data for generative AI, The Govlab has defined five scenarios outlining five different ways in which open data and generative AI can intersect. These scenarios are intended as a starting point, to be expanded in the future, based on available use cases.
Scenario |
Function |
Quality requirements |
Metadata requirements |
Example |
Pre-training |
Training the foundational layers of a generative AI model with large amounts of open data. |
High volume of data, diverse and representative of the application domain and non-structured usage. |
Clear information on the source of the data. |
Data from NASA's Harmonized Landsat Sentinel-2 (HLS) project were used to train the geospatial foundational model watsonx.ai. |
Adaptation |
Refinement of a pre-trained model with task-specific open data, using fine-tuning or RAG techniques. |
Tabular and/or unstructured data of high accuracy and relevance to the target task, with a balanced distribution. |
Metadata focused on the annotation and provenance of data to provide contextual enrichment. |
Building on the LLaMA 70B model, the French Government created LLaMandement, a refined large language model for the analysis and drafting of legal project summaries. They used data from SIGNALE, the French government's legislative platform. |
Inference and Insight Generation |
Extracting information and patterns from open data using a trained generative AI model. |
High quality, complete and consistent tabular data. |
Descriptive metadata on the data collection methods, source information and version control. |
Wobby is a generative interface that accepts natural language queries and produces answers in the form of summaries and visualisations, using datasets from different offices such as Eurostat or the World Bank. |
Data Augmentation |
Leveraging open data to generate synthetic data or provide ontologies to extend the amount of training data. |
Tabular and/or unstructured data which is a close representation of reality, ensuring compliance with ethical considerations. |
Transparency about the generation process and possible biases. |
A team of researchers adapted the US Synthea model to include demographic and hospital data from Australia. Using this model, the team was able to generate approximately 117,000 region-specific synthetic medical records. |
Open-Ended Exploration |
Exploring and discovering new knowledge and patterns in open data through generative models. |
Tabular data and/or unstructured, diverse and comprehensive. |
Clear information on sources and copyright, understanding of possible biases and limitations, identification of entities. |
NEPAccess is a pilot to unlock access to data related to the US National Environmental Policy Act (NEPA) through a generative AI model. It will include functions for drafting environmental impact assessments, data analysis, etc. |
Figure 2. Five scenarios where open data and Artificial Intelligence converge, adapted from the report “A Fourth Wave of Open Data? Exploring the Spectrum of Scenarios for Open Data and Generative AI”, The Govlab, 2024.
You can read the details of these scenarios in the report, where more examples are explained. In addition, The Govlab has also launched an observatory where it collects examples of intersections between open data and generative artificial intelligence. It includes the examples in the report along with additional examples. Any user can propose new examples via this form. These examples will be used to further study the field and improve the scenarios currently defined.
Among the cases that can be seen on the web, we find a Spanish company: Tendios. This is a software-as-a-service company that has developed a chatbot to assist in the analysis of public tenders and bids in order to facilitate competition. This tool is trained on public documents from government tenders.
Recommendations for data publishers
To extract the full potential of generative AI, improving its efficiency and effectiveness, the report highlights that open data providers need to address a number of challenges, such as improving data governance and management. In this regard, they contain five recommendations:
- Improve transparency and documentation. Through the use of standards, data dictionaries, vocabularies, metadata templates, etc. It will help to implement documentation practices on lineage, quality, ethical considerations and impact of results.
- Maintaining quality and integrity. Training and routine quality assurance processes are needed, including automated or manual validation, as well as tools to update datasets quickly when necessary. In addition, mechanisms for reporting and addressing data-related issues that may arise are needed to foster transparency and facilitate the creation of a community around open datasets.
- Promote interoperability and standards. It involves adopting and promoting international data standards, with a special focus on synthetic data and AI-generated content.
- Improve accessibility and user-friendliness. It involves the enhancement of open data portals through intelligent search algorithms and interactive tools. It is also essential to establish a shared space where data publishers and users can exchange views and express needs in order to match supply and demand.
- Addressing ethical considerations. Protecting data subjects is a top priority when talking about open data and generative AI. Comprehensive ethics committees and ethical guidelines are needed around the collection, sharing and use of open data, as well as advanced privacy-preserving technologies.
This is an evolving field that needs constant updating by data publishers. These must provide technically and ethically adequate datasets for generative AI systems to reach their full potential.