What data governance should look like in open source AI models
Fecha de la noticia: 17-02-2025
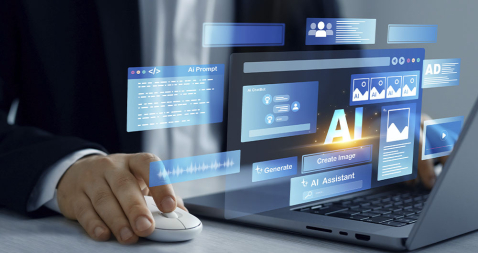
Open source artificial intelligence (AI) is an opportunity to democratise innovation and avoid the concentration of power in the technology industry. However, their development is highly dependent on the availability of high quality datasets and the implementation of robust data governance frameworks. A recent report by Open Future and the Open Source Initiative (OSI) analyses the challenges and opportunities at this intersection, proposing solutions for equitable and accountable data governance. You can read the full report here.
In this post, we will analyse the most relevant ideas of the document, as well as the advice it offers to ensure a correct and effective data governance in artificial intelligence open source and take advantage of all its benefits.
The challenges of data governance in AI
Despite the vast amount of data available on the web, accessing and using it to train AI models poses significant ethical, legal and technical challenges. For example:
- Balancing openness and rights: In line with the Data Governance Regulation (DGA), broad access to data should be guaranteed without compromising intellectual property rights, privacy and fairness.
- Lack of transparency and openness standards: It is important that models labelled as "open" meet clear criteria for transparency in the use of data.
- Structural biases: Many datasets reflect linguistic, geographic and socio-economic biases that can perpetuate inequalities in AI systems.
- Environmental sustainability: the intensive use of resources to train AI models poses sustainability challenges that must be addressed with more efficient practices.
- Engage more stakeholders: Currently, developers and large corporations dominate the conversation on AI, leaving out affected communities and public organisations.
Having identified the challenges, the report proposes a strategy for achieving the main goal: adequate data governance in open source AI models. This approach is based on two fundamental pillars.
Towards a new paradigm of data governance
Currently, access to and management of data for training AI models is marked by increasing inequality. While some large corporations have exclusive access to vast data repositories, many open source initiatives and marginalised communities lack the resources to access quality, representative data. To address this imbalance, a new approach to data management and use in open source AI is needed. The report highlights two fundamental changes in the way data governance is conceived:
On the one hand, adopting a data commons approach which is nothing more than an access model that ensures a balance between data openness and rights protection.. To this end, it would be important to use innovative licences that allow data sharing without undue exploitation. It is also relevant to create governance structures that regulate access to and use of data. And finally, implement compensation mechanisms for communities whose data is used in artificial intelligence.
On the other hand, it is necessary to transcend the vision focused on AI developers and include more actors in data governance, such as:
- Data owners and content-generating communities.
- Public institutions that can promote openness standards.
- Civil society organisations that ensure fairness and responsible access to data.
By adopting these changes, the AI community will be able to establish a more inclusive system, in which the benefits of data access are distributed in a manner that is equitable and respectful of the rights of all stakeholders. According to the report, the implementation of these models will not only increase the amount of data available for open source AI, but will also encourage the creation of fairer and more sustainable tools for society as a whole.
Advice and strategy
To make robust data governance effective in open source AI, the report proposes six priority areas for action:
- Data preparation and traceability: Improve the quality and documentation of data sets.
- Licensing and consent mechanisms: allow data creators to clearly define their use.
- Data stewardship: strengthen the role of intermediaries who manage data ethically.
- Environmental sustainability: Reduce the impact of AI training with efficient practices.
- Compensation and reciprocity: ensure that the benefits of AI reach those who contribute data.
- Public policy interventions: promote regulations that encourage transparency and equitable access to data.
Open source artificial intelligence can drive innovation and equity, but to achieve this requires a more inclusive and sustainable approach to data governance. Adopting common data models and broadening the ecosystem of actors will build AI systems that are fairer, more representative and accountable to the common good.
The report published by Open Future and Open Source Initiative calls for action from developers, policymakers and civil society to establish shared standards and solutions that balance open data with the protection of rights. With strong data governance, open source AI will be able to deliver on its promise to serve the public interest.