Podcast: How to learn data science in a self-taught way
Fecha: 31-03-2025
Nombre: Juan Benavente, ingeniero industrial y científico de datos y Alejandro Alija, doctor en física, científico de datos y experto en transformación digital
Sector: Science and technology, Education
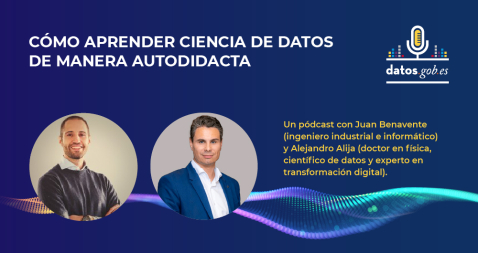
Did you know that data science skills are among the most in-demand skills in business? In this podcast, we are going to tell you how you can train yourself in this field, in a self-taught way. For this purpose, we will have two experts in data science:
- Juan Benavente, industrial and computer engineer with more than 12 years of experience in technological innovation and digital transformation. In addition, it has been training new professionals in technology schools, business schools and universities for years.
- Alejandro Alija, PhD in physics, data scientist and expert in digital transformation. In addition to his extensive professional experience focused on the Internet of Things (internet of things), Alejandro also works as a lecturer in different business schools and universities.
Listen to the podcast (in spanish)
Summary of the interview
-
What is data science? Why is it important and what can it do for us?
Alejandro Alija: Data science could be defined as a discipline whose main objective is to understand the world, the processes of business and life, by analysing and observing data.Data science is a discipline whose main objective is to understand the world, the processes of business and life, by analysing and observing the data.. In the last 20 years it has gained exceptional relevance due to the explosion in data generation, mainly due to the irruption of the internet and the connected world.
Juan Benavente: The term data science has evolved since its inception. Today, a data scientist is the person who is working at the highest level in data analysis, often associated with the building of machine learning or artificial intelligence algorithms for specific companies or sectors, such as predicting or optimising manufacturing in a plant.
The profession is evolving rapidly, and is likely to fragment in the coming years. We have seen the emergence of new roles such as data engineers or MLOps specialists. The important thing is that today any professional, regardless of their field, needs to work with data. There is no doubt that any position or company requires increasingly advanced data analysis. It doesn't matter if you are in marketing, sales, operations or at university. Anyone today is working with, manipulating and analysing data. If we also aspire to data science, which would be the highest level of expertise, we will be in a very beneficial position. But I would definitely recommend any professional to keep this on their radar.
-
How did you get started in data science and what do you do to keep up to date? What strategies would you recommend for both beginners and more experienced profiles?
Alejandro Alija: My basic background is in physics, and I did my PhD in basic science. In fact, it could be said that any scientist, by definition, is a data scientist, because science is based on formulating hypotheses and proving them with experiments and theories. My relationship with data started early in academia. A turning point in my career was when I started working in the private sector, specifically in an environmental management company that measures and monitors air pollution. The environment is a field that is traditionally a major generator of data, especially as it is a regulated sector where administrations and private companies are obliged, for example, to record air pollution levels under certain conditions. I found historical series up to 20 years old that were available for me to analyse. From there my curiosity began and I specialised in concrete tools to analyse and understand what is happening in the world.
Juan Benavente: I can identify with what Alejandro said because I am not a computer scientist either. I trained in industrial engineering and although computer science is one of my interests, it was not my base. In contrast, nowadays, I do see that more specialists are being trained at the university level. A data scientist today has manyskills on their back such as statistics, mathematics and the ability to understand everything that goes on in the industry. I have been acquiring this knowledge through practice. On how to keep up to date, I think that, in many cases, you can be in contact with companies that are innovating in this field. A lot can also be learned at industry or technology events. I started in the smart cities and have moved on to the industrial world to learn little by little.
Alejandro Alija:. To add another source to keep up to date. Apart from what Juan has said, I think it's important to identify what we call outsiders, the manufacturers of technologies, the market players. They are a very useful source of information to stay up to date: identify their futures strategies and what they are betting on.
-
If someone with little or no technical knowledge wants to learn data science, where do they start?
Juan Benavente: In training, I have come across very different profiless: from people who have just graduated from university to profiles that have been trained in very different fields and find in data science an opportunity to transform themselves and dedicate themselves to this. Thinking of someone who is just starting out, I think the best thing to do is put your knowledge into practice. In projects I have worked on, we defined the methodology in three phases: a first phase of more theoretical aspects, taking into account mathematics, programming and everything a data scientist needs to know; once you have those basics, the sooner you start working and practising those skills, the better. I believe that skill sharpens the wit and, both to keep up to date and to train yourself and acquire useful knowledge, the sooner you enter into a project, the better. And even more so in a world that is so frequently updated. In recent years, the emergence of the Generative AI has brought other opportunities. There are also opportunities for new profiles who want to be trained . Even if you are not an expert in programming, you have tools that can help you with programming, and the same can happen in mathematics or statistics.
Alejandro Alija:. To complement what Juan says from a different perspective. I think it is worth highlighting the evolution of the data science profession.. I remember when that paper about "the sexiest profession in the world" became famous and went viral, but then things adjusted. The first settlers in the world of data science did not come so much from computer science or informatics. There were more outsiders: physicists, mathematicians, with a strong background in mathematics and physics, and even some engineers whose work and professional development meant that they ended up using many tools from the computer science field. Gradually, it has become more and more balanced. It is now a discipline that continues to have those two strands: people who come from the world of physics and mathematics towards the more basic data, and people who come with programming skills. Everyone knows what they have to balance in their toolbox. Thinking about a junior profile who is just starting out, I think a very important thing - and we see this when we teach - is programming skills. I would say that having programming skills is not just a plus, but a basic requirement for advancement in this profession. It is true that some people can do well without a lot of programming skills, but I would argue that a beginner needs to have those first programming skills with a basic toolset . We're talking about languages such as Python and R, which are the headline languages. You don't need to be a great coder, but you do need to have some basic knowledge to get started. Then, of course, specific training in the mathematical foundations of data science is crucial. The fundamental statistics and more advanced statistics are complements that, if present, will move a person along the data science learning curve much faster. Thirdly, I would say that specialisation in particular tools is important. Some people are more oriented towards data engineering, others towards the modelling world. Ideally, specialise in a few frameworks and use them together, as optimally as possible.
-
In addition to teaching, you both work in technology companies. What technical certifications are most valued in the business sector and what open sources of knowledge do you recommend to prepare for them?
Juan Benavente: Personally, it's not what I look at most, but I think it can be relevant, especially for people who are starting out and need help in structuring their approach to the problem and understanding it. I recommend certifications of technologies that are in use in any company where you want to end up working. Especially from providers of cloud computing and widespread data analytics tools. These are certifications that I would recommend for someone who wants to approach this world and needs a structure to help them. When you don't have a knowledge base, it can be a bit confusing to understand where to start. Perhaps you should reinforce programming or mathematical knowledge first, but it can all seem a bit complicated. Where these certifications certainly help you is, in addition to reinforcing concepts, to ensure that you are moving well and know the typical ecosystem of tools you will be working with tomorrow. It is not just about theoretical concepts, but about knowing the ecosystems that you will encounter when you start working, whether you are starting your own company or working in an established company. It makes it much easier for you to get to know the typical ecosystem of tools. Call it Microsoft Computing, Amazon or other providers of such solutions. This will allow you to focus more quickly on the work itself, and less on all the tools that surround it. I believe that this type of certification is useful, especially for profiles that are approaching this world with enthusiasm. It will help them both to structure themselves and to land well in their professional destination. They are also likely to be valued in selection processes.
Alejandro Alija: If someone listens to us and wants more specific guidelines, it could be structured in blocks. There are a series of massive online courses that, for me, were a turning point. In my early days, I tried to enrol in several of these courses on platforms such as Coursera, edX, where even the technology manufacturers themselves design these courses. I believe that this kind of massive, self-service, online courses provide a good starting base. A second block would be the courses and certifications of the big technology providers, such as Microsoft, Amazon Web Services, Google and other platforms that are benchmarks in the world of data. These companies have the advantage that their learning paths are very well structured, which facilitates professional growth within their own ecosystems. Certifications from different suppliers can be combined. For a person who wants to go into this field, the path ranges from the simplest to the most advanced certifications, such as being a data solutions architect or a specialist in a specific data analytics service or product. These two learning blocks are available on the internet, most of them are open and free or close to free. Beyond knowledge, what is valued is certification, especially in companies looking for these professional profiles.
-
In addition to theoretical training, practice is key, and one of the most interesting methods of learning is to replicate exercises step by step. In this sense, from datos.gob.es we offer didactic resources, many of them developed by you as experts in the project, can you tell us what these exercises consist of?. How are they approached?
Alejandro Alija: The approach we always took was designed for a broad audience, without complex prerequisites. We wanted any user of the portal to be able to replicate the exercises, although it is clear that the more knowledge you have, the more you can use it to your advantage. Exercises have a well-defined structure: a documentary section, usually a content post or a report describing what the exercise consists of, what materials are needed, what the objectives are and what it is intended to achieve. In addition, we accompany each exercise with two additional resources. The first resource is a code repository where we upload the necessary materials, with a brief description and the code of the exercise. It can be a Python notebook , a Jupyter Notebook or a simple script, where the technical content is. And then another fundamental element that we believe is important and that is aimed at facilitating the execution of the exercises. In data science and programming, non-specialist users often find it difficult to set up a working environment. A Python exercise, for example, requires having a programming environment installed, knowing the necessary libraries and making configurations that are trivial for professionals, but can be very complex for beginners. To mitigate this barrier, we publish most of our exercises on Google Colab, a wonderful and open tool. Google Colab is a web programming environment where the user only needs a browser to access it. Basically, Google provides us with a virtual computer where we can run our programmes and exercises without the need for special configurations. The important thing is that the exercise is ready to use and we always check it in this environment, which makes it much easier to learn for beginners or less technically experienced users.
Juan Benavente: Yes, we always take a user-oriented approach, step by step, trying to make it open and accessible. The aim is for anyone to be able to run an exercise without the need for complex configurations, focusing on topics as close to reality as possible. We often take advantage of open data published by entities such as the DGT or other bodies to make realistic analyses. We have developed very interesting exercises, such as energy market predictions, analysis of critical materials for batteries and electronics, which allow learning not only about technology, but also about the specific subject matter.. You can get down to work right away, not only to learn, but also to find out about the subject.
-
In closing, we'd like you to offer a piece of advice that is more attitude-oriented than technical, what would you say to someone starting out in data science?
Alejandro Alija: As for an attitude tip for someone starting out in data science, I suggest be brave. There is no need to worry about being unprepared, because in this field everything is to be done and anyone can contribute value. Data science is multi-faceted: there are professionals closer to the business world who can provide valuable insights, and others who are more technical and need to understand the context of each area. My advice is to be content with the resources available without panicking, because, although the path may seem complex, the opportunities are very high. As a technical tip, it is important to be sensitive to the development and use of data. The more understanding one has of this world, the smoother the approach to projects will be.
Juan Benavente: I endorse the advice to be brave and add a reflection on programming: many people find the theoretical concept attractive, but when they get to practice and see the complexity of programming, some are discouraged by lack of prior knowledge or different expectations. It is important to add the concepts of patience and perseverance. When you start in this field, you are faced with multiple areas that you need to master: programming, statistics, mathematics, and specific knowledge of the sector you will be working in, be it marketing, logistics or another field. The expectation of becoming an expert quickly is unrealistic. It is a profession that, although it can be started without fear and by collaborating with professionals, requires a journey and a learning process. You have to be consistent and patient, managing expectations appropriately. Most people who have been in this world for a long time agree that they have no regrets about going into data science. It is a very attractive profession where you can add significant value, with an important technological component. However, the path is not always straightforward. There will be complex projects, moments of frustration when analyses do not yield the expected results or when working with data proves more challenging than expected. But looking back, few professionals regret having invested time and effort in training and developing in this field. In summary, the key tips are: courage to start, perseverance in learning and development of programming skills.