Invisibilisation and algorithmic discrimination
Fecha de la noticia: 06-10-2023
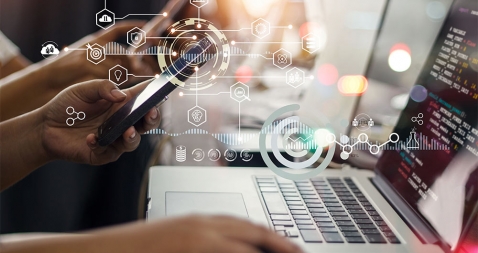
Digital technology and algorithms have revolutionised the way we live, work and communicate. While promising efficiency, accuracy and convenience, these technologies can exacerbate prejudice and social inequalities exacerbate prejudice and social inequalities and create new forms of exclusion and create new forms of exclusion. Thus, invisibilisation and discrimination, which have always existed, take on new forms in the age of algorithms.
Lack of interest and data leads to algorithmic invisibilisation, leading to two types of algorithmic neglect. The first of these is among the world's underserved, which includes the millions who do not have a smartphone or a bank account millions who do not have a smartphone or a bank account, and are thus on the margins of the platform economy and who are therefore on the margins of the platform economy and, for algorithms, do not exist. The second type of algorithmic abandonment includes individuals or groups who are victims of the failure of the algorithmic system, as was the case with SyRI(Systeem Risico Indicatie)SyRI(Systeem Risico Indicatie) in the Netherlands that unfairly singled out some 20,000 families from low socio-economic backgrounds for tax fraud, leading many to ruin by 2021. The algorithm, which the algorithm, which was declared illegal by a court in The Hague months later, was applied in the country's poorest neighbourhoodsthe algorithm, which was declared illegal by a court in The Hague months later, was applied in the country's poorest neighbourhoods the algorithm, which was declared illegal by a court in The Hague months later, was applied in the poorest neighbourhoods of the country and blocked many families with more than one nationality from receiving the social benefits to which they were entitled because of their socio-economic status.
Beyond the example in the Dutch public system, invisibilisation and discrimination can also originate in the private sector. One example is Amazon's amazon's job posting algorithm which showed a bias against women by learning from historical data - i.e. incomplete data because it did not include a large and representative universe - leading Amazon to abandon the project. which showed a bias against women by learning from historical data - i.e. incomplete data because it did not include a large and representative universe - leading Amazon to abandon the project. Another example is Apple Card, a credit card backed by Goldman Sachs, which was also singled out when its algorithm was found to offer more favourable credit limits to men than to women.
In general, invisibility and algorithmic discrimination, in any field, can lead to unequal access to resources and exacerbate social and economic exclusion.
Making decisions based on algorithms
Data and algorithms are interconnected components in computing and information processing. Data serve as a basis, but can be unstructured, with excessive variability and incompleteness. Algorithms are instructions or procedures designed to process and structure this data and extract meaningful information, patterns or results.
The quality and relevance of the data directly impacts the effectiveness of the algorithms, as they rely on the data inputs to generate results. Hence, the principle "rubbish in, rubbish out"which summarises the idea that if poor quality, biased or inaccurate data enters a system or process, the result will also be of poor quality or inaccurate. On the other hand, well-designed well-designed algorithms can enhance the value of data by revealing hidden relationships or making by revealing hidden relationships or making predictions.
This symbiotic relationship underscores the critical role that both data and algorithms play in driving technological advances, enabling informed decision making, and This symbiotic relationship underscores the critical role that both data and algorithms play in driving technological advances, enabling informed decision making, and fostering innovation.
Algorithmic decision making refers to the process of using predefined sets of instructions or rules to analyse data and make predictions to aid decision making. Increasingly, it is being applied to decisions that have to do with social welfare social welfare and the provision of commercial services and products through platforms. This is where invisibility or algorithmic discrimination can be found.
Increasingly, welfare systems are using data and algorithms to help make decisions on issues such as who should receive what kind of care and who is at risk. These algorithms consider different factors such as income, family or household size, expenditures, risk factors, age, sex or gender, which may include biases and omissions.
That is why the That is why the Special Rapporteur on extreme poverty and human rights, Philip Alston, warned in a report to the UN General Assembly that the uncautious adoption of these can lead to dystopian social welfare dystopian social welfare. In such a dystopian welfarestate , algorithms are used to reduce budgets, reduce the number of beneficiaries, eliminate services, introduce demanding and intrusive forms of conditionality, modify behaviour, impose sanctions and "reverse the notion that the state is accountable".
Algorithmic invisibility and discrimination: Two opposing concepts
Although data and algorithms have much in common, algorithmic invisibility and discrimination are two opposing concepts. Algorithmic invisibility refers to gaps in data sets or omissions in algorithms, which result in inattentions in the application of benefits or services. In contrast, algorithmic discrimination speaks to hotspots that highlight specific communities or biased characteristics in datasets, generating unfairness.
That is, algorithmic invisibilisation occurs when individuals or groups are absent from datasets, making it impossible to address their needs. For example, integrating data on women with disabilities into social decision-making can be vital for the inclusion of women with disabilities in society. Globally, women are more vulnerable to algorithmic invisibilisation than men, as they have less access to digital technology have less access to digital technology and leave fewer digital traces.
Opaque algorithmic systems that incorporate stereotypes can increase invisibilisation and discrimination by hiding or targeting vulnerable individuals or populations. An opaque algorithmic system is one that does not allow access to its operation.
On the other hand, aggregating or disaggregating data without careful consideration of the consequences can result in omissions or errors result in omissions or errors. This illustrates the double-edged nature of accounting; that is, the ambivalence of technology that quantifies and counts, and that can serve to improve people's lives, but also to harm them.
Discrimination can arise when algorithmic decisions are based on historical data, which usually incorporate asymmetries, stereotypes and injustices, because more inequalities existed in the past. The "rubbish in, rubbish out" effect occurs if the data is skewed, as is often the case with online content. Also, biased or incomplete databases can be incentives for algorithmic discrimination. Selection biases may arise when facial recognition data, for example, is based on the features of white men, while the users are dark-skinned women, or on online content generated by a minority of agentswhich makes generalisation difficult.
As can be seen, tackling invisibility and algorithmic discrimination is a major challenge that can only be solved through awareness-raising and collaboration between institutions, campaigning organisations, businesses and research.
Content prepared by Miren Gutiérrez, PhD and researcher at the University of Deusto, expert in data activism, data justice, data literacy and gender disinformation.
The contents and views reflected in this publication are the sole responsibility of the author.