Synthetic Data - What are they? And what are they used for?
Fecha del documento: 17-10-2023
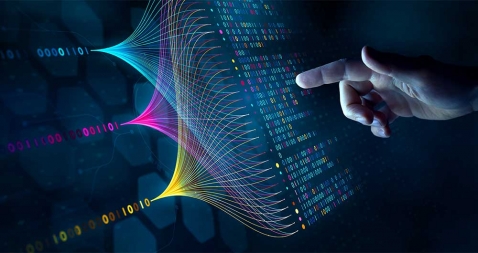
In the era of data, we face the challenge of a scarcity of valuable data for building new digital products and services. Although we live in a time when data is everywhere, we often struggle to access quality data that allows us to understand processes or systems from a data-driven perspective. The lack of availability, fragmentation, security, and privacy are just some of the reasons that hinder access to real data.
However, synthetic data has emerged as a promising solution to this problem. Synthetic data is artificially created information that mimics the characteristics and distributions of real data, without containing personal or sensitive information. This data is generated using algorithms and techniques that preserve the structure and statistical properties of the original data.
Synthetic data is useful in various situations where the availability of real data is limited or privacy needs to be protected. It has applications in scientific research, software and system testing, and training artificial intelligence models. It enables researchers to explore new approaches without accessing sensitive data, developers to test applications without exposing real data, and AI experts to train models without the need to collect all the real-world data, which is sometimes simply impossible to capture within reasonable time and cost.
There are different methods for generating synthetic data, such as resampling, probabilistic and generative modeling, and perturbation and masking methods. Each method has its advantages and challenges, but overall, synthetic data offers a secure and reliable alternative for analysis, experimentation, and AI model training.
It is important to highlight that the use of synthetic data provides a viable solution to overcome limitations in accessing real data and address privacy and security concerns. Synthetic data allows for testing, algorithm training, and application development without exposing confidential information. However, ensuring the quality and fidelity of synthetic data is crucial through rigorous evaluations and comparisons with real data.
In this report, we provide an introductory overview of the discipline of synthetic data, illustrating some valuable use cases for different types of synthetic data that can be generated. Autonomous vehicles, DNA sequencing, and quality controls in production chains are just a few of the cases detailed in this report. Furthermore, we highlight the use of the open-source software SDV (Synthetic Data Vault), developed in the academic environment of MIT, which utilizes machine learning algorithms to create tabular synthetic data that imitates the properties and distributions of real data. We present a practical example in a Google Colab environment to generate synthetic data about fictional customers hosted in a fictional hotel. We follow a workflow that involves preparing real data and metadata, training the synthesizer, and generating synthetic data based on the learned patterns. Additionally, we apply anonymization techniques to protect sensitive data and evaluate the quality of the generated synthetic data.
In summary, synthetic data is a powerful tool in the data era, as it allows us to overcome the scarcity and lack of availability of valuable data. With its ability to mimic real data without compromising privacy, synthetic data has the potential to transform the way we develop AI projects and conduct analysis. As we progress in this new era, synthetic data is likely to play an increasingly important role in generating new digital products and services.
If you want to know more about the content of this report, you can watch the interview with its author.
Below, you can download the full report, the executive summary and a presentation-summary.