Data Sandboxes: Exploring the potential of open data in a secure environment
Fecha de la noticia: 27-09-2024
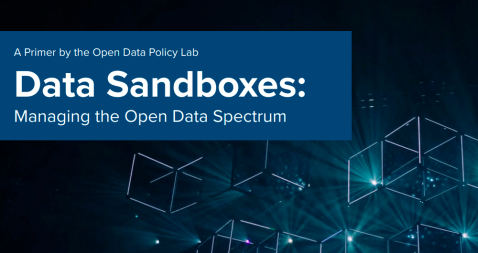
Data sandboxes are tools that provide us with environments to test new data-related practices and technologies, making them powerful instruments for managing and using data securely and effectively. These spaces are very useful in determining whether and under what conditions it is feasible to open the data. Some of the benefits they offer are:
- Controlled and secure environments: provide a workspace where information can be explored and its usefulness and quality assessed before committing to wider sharing. This is particularly important in sensitive sectors, where privacy and data security are paramount.
- Innovation: they provide a safe space for experimentation and rapid prototyping, allowing for rapid iteration, testing and refining new ideas and data-driven solutions as test bench before launching them to the public.
- Multi-sectoral collaboration: facilitate collaboration between diverse actors, including government entities, private companies, academia and civil society. This multi-sectoral approach helps to break down data silos and promotes the sharing of knowledge and good practices across sectors.
- Adaptive and scalable use: they can be adjusted to suit different data types, use cases and sectors, making them a versatile tool for a variety of data-driven initiatives.
- Cross-border data exchange: they provide a viable solution to manage the challenges of data exchange between different jurisdictions, especially with regard to international privacy regulations.
The report "Data Sandboxes: Managing the Open Data Spectrum" explores the concept of data sandboxes as a tool to strike the right balance between the benefits of open data and the need to protect sensitive information.
Value proposition for innovation
In addition to all the benefits outlined above, data sandboxes also offer a strong value proposition for organisations looking to innovate responsibly. These environments help us to improve data quality by making it easier for users to identify inconsistencies so that improvements can be made. They also contribute to reducing risks by providing secure environments to enable work with sensitive data. By fostering cross-disciplinary experimentation, collaboration and innovation, they contribute to increasing the usability of data and developing a data-driven culture within organisations. In addition, data sandboxes help reduce barriers to data access , improving transparency and accountability, which strengthens citizens' trust and leads to an expansion of data exchanges.
Types of data sandboxes and characteristics
Depending on the main objective when implementing a sandbox, there are three different types of sandboxes:
- Regulatory sandboxes, which allow companies and organisations to test innovative services under the close supervision of regulators in a specific sector or area.
- Innovation sandboxes, which are frequently used by developers to test new features and get quick feedback on their work.
- Research sandboxes, which make it easier for academia and industry to safely test new algorithms or models by focusing on the objective of their tests, without having to worry about breaching established regulations.
In any case, regardless of the type of sandbox we are working with, they are all characterised by the following common key aspects:
Figure 1. Characteristics of a data sandbox. Adaptation of a visual of The Govlab.
Each of these is described below:
- Controlled: these are restricted environments where sensitive and analysed data can be accessed securely, ensuring compliance with relevant regulations.
- Secure: they protect the privacy and security of data, often using anonymised or synthetic data.
- Collaborative: facilitating collaboration between different regions, sectors and roles, strengthening data ecosystems.
- High computational capacity: provide advanced computational resources capable of performing complex tasks on the data when needed.
- Temporal in nature: They are designed for temporary use and with a short life cycle, allowing for rapid and focused experimentation that either concludes once its objective is achieved or becomes a new long-term project.
- Adaptable: They are flexible enough to customise and scale according to needs and different data types, use cases and contexts.
Examples of data sandboxes
Data sandboxes have long been successfully implemented in multiple sectors across Europe and around the world, so we can easily find several examples of their implementation on our continent:
- Data science lab in Denmark: it provides access to sensitive administrative data useful for research, fostering innovation under strict data governance policies.
- TravelTech in Lithuania: an open access sandbox that provides tourism data to improve business and workforce development in the sector.
- INDIGO Open Data Sandbox: it promotes data sharing across sectors to improve social policies, with a focus on creating a secure environment for bilateral data sharing initiatives.
- Health data science sandbox in Denmark: a training platform for researchers to practice data analysis using synthetic biomedical data without having to worry about strict regulation.
Future direction and challenges
As we have seen, data sandboxes can be a powerful tool for fostering open data, innovation and collaboration, while ensuring data privacy and security. By providing a controlled environment for experimentation with data, they enable all interested parties to explore new applications and knowledge in a reliable and safe way. Sandboxes can therefore help overcome initial barriers to data access and contribute to fostering a more informed and purposeful use of data, thus promoting the use of data-driven solutions to public policy problems.
However, despite their many benefits, data sandboxes also present a number of implementation challenges. The main problems we might encounter in implementing them include:
- Relevance: ensure that the sandbox contains high quality and relevant data, and that it is kept up to date.
- Governance: establish clear rules and protocols for data access, use and sharing, as well as monitoring and compliance mechanisms.
- Scalability: successfully export the solutions developed within the sandbox and be able to translate them into practical applications in the real world.
- Risk management: address comprehensively all risks associated with the re-use of data throughout its lifecycle and without compromising its integrity.
However, as technologies and policies continue to evolve, it is clear that data sandboxes are set to be a useful tool and play an important role in managing the spectrum of data openness, thereby driving the use of data to solve increasingly complex problems. Furthermore, the future of data sandboxes will be influenced by new regulatory frameworks (such as Data Regulations and Data Governance) that reinforce data security and promote data reuse, and by integration with privacy preservation and privacy enhancing technologies that allow us to use data without exposing any sensitive information. Together, these trends will drive more secure data innovation within the environments provided by data sandboxes.
Content prepared by Carlos Iglesias, Open data Researcher and consultant, World Wide Web Foundation. The contents and views expressed in this publication are the sole responsibility of the author.