The role of open data in the evolution of SLM and LLM: efficiency vs. power
Fecha de la noticia: 27-12-2024
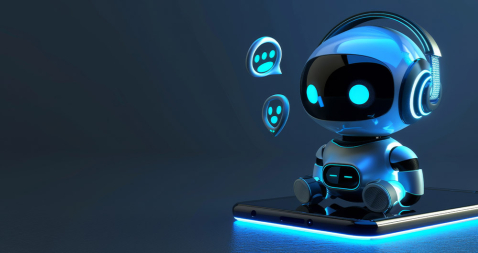
Language models are at the epicentre of the technological paradigm shift that has been taking place in generative artificial intelligence (AI) over the last two years. From the tools with which we interact in natural language to generate text, images or videos and which we use to create creative content, design prototypes or produce educational material, to more complex applications in research and development that have even been instrumental in winning the 2024 Nobel Prize in Chemistry, language models are proving their usefulness in a wide variety of applicationsthat we are still exploring.
Since Google's influential 2017 paper "Attention is all you need" describing the architecture of the Transformers, the technology underpinning the new capabilities that OpenAI popularised in late 2022 with the launch of ChatGPT, the evolution of language models has been more than dizzying. In just two years, we have moved from models focused solely on text generation to multimodal versions that integrate interaction and generation of text, images and audio.
This rapid evolution has given rise to two categories of language models: SLMs (Small Language Models), which are lighter and more efficient, and LLLMs (Large Language Models), which are heavier and more powerful. Far from considering them as competitors, we should analyse SLM and LLM as complementary technologies. While LLLMs offer general processing and content generation capabilities, SLMs can provide support for more agile and specialised solutions for specific needs. However, both share one essential element: they rely on large volumes of data for training and at the heart of their capabilities is open data, which is part of the fuel used to train these language models on which generative AI applications are based.
LLLM: power driven by massive data
The LLLMs are large-scale language models with billions, even trillions, of parameters. These parameters are the mathematical units that allow the model to identify and learn patterns in the training data, giving them an extraordinary ability to generate text (or other formats) that is consistent and adapted to the users' context. These models, such as the GPT family from OpenAI, Gemini from Google or Llama from Meta, are trained on immense volumes of data and are capable of performing complex tasks, some even for which they were not explicitly trained.
Thus, LLMs are able to perform tasks such as generating original content, answering questions with relevant and well-structured information or generating software code, all with a level of competence equal to or higher than humans specialised in these tasks and always maintaining complex and fluent conversations.
The LLLMs rely on massive amounts of data to achieve their current level of performance: from repositories such as Common Crawl, which collects data from millions of web pages, to structured sources such as Wikipedia or specialised sets such as PubMed Open Access in the biomedical field. Without access to these massive bodies of open data, the ability of these models to generalise and adapt to multiple tasks would be much more limited.
However, as LLMs continue to evolve, the need for open data increases to achieve specific advances such as:
- Increased linguistic and cultural diversity: although today's LLMs are multilingual, they are generally dominated by data in English and other major languages. The lack of open data in other languages limits the ability of these models to be truly inclusive and diverse. More open data in diverse languages would ensure that LLMs can be useful to all communities, while preserving the world's cultural and linguistic richness.
- Reducción de sesgos: los LLM, como cualquier modelo de IA, son propensos a reflejar los sesgos presentes en los datos con los que se entrenan. This sometimes leads to responses that perpetuate stereotypes or inequalities. Incorporating more carefully selected open data, especially from sources that promote diversity and equality, is fundamental to building models that fairly and equitably represent different social groups.
- Constant updating: Data on the web and other open resources is constantly changing. Without access to up-to-date data, the LLMs generate outdated responses very quickly. Therefore, increasing the availability of fresh and relevant open data would allow LLMs to keep in line with current events[9].
- Entrenamiento más accesible: a medida que los LLM crecen en tamaño y capacidad, también lo hace el coste de entrenarlos y afinarlos. Open data allows independent developers, universities and small businesses to train and refine their own models without the need for costly data acquisitions. This democratises access to artificial intelligence and fosters global innovation.
To address some of these challenges, the new Artificial Intelligence Strategy 2024 includes measures aimed at generating models and corpora in Spanish and co-official languages, including the development of evaluation datasets that consider ethical evaluation.
SLM: optimised efficiency with specific data
On the other hand, SLMs have emerged as an efficient and specialised alternative that uses a smaller number of parameters (usually in the millions) and are designed to be lightweight and fast. Aunque no alcanzan la versatilidad y competencia de los LLM en tareas complejas, los SLM destacan por su eficiencia computacional, rapidez de implementación y capacidad para especializarse en dominios concretos.
For this, SLMs also rely on open data, but in this case, the quality and relevance of the datasets are more important than their volume, so the challenges they face are more related to data cleaning and specialisation. These models require sets that are carefully selected and tailored to the specific domain for which they are to be used, as any errors, biases or unrepresentativeness in the data can have a much greater impact on their performance. Moreover, due to their focus on specialised tasks, the SLMs face additional challenges related to the accessibility of open data in specific fields. For example, in sectors such as medicine, engineering or law, relevant open data is often protected by legal and/or ethical restrictions, making it difficult to use it to train language models.
The SLMs are trained with carefully selected data aligned to the domain in which they will be used, allowing them to outperform LLMs in accuracy and specificity on specific tasks, such as for example:
- Text autocompletion: a SLM for Spanish autocompletion can be trained with a selection of books, educational texts or corpora such as those to be promoted in the aforementioned AI Strategy, being much more efficient than a general-purpose LLM for this task.
- Legal consultations: a SLM trained with open legal datasets can provide accurate and contextualised answers to legal questions or process contractual documents more efficiently than a LLM.
- Customised education: ein the education sector, SLM trained with open data teaching resources can generate specific explanations, personalised exercises or even automatic assessments, adapted to the level and needs of the student.
- Medical diagnosis: An SLM trained with medical datasets, such as clinical summaries or open publications, can assist physicians in tasks such as identifying preliminary diagnoses, interpreting medical images through textual descriptions or analysing clinical studies.
Ethical Challenges and Considerations
We should not forget that, despite the benefits, the use of open data in language modelling presents significant challenges. One of the main challenges is, as we have already mentioned, to ensure the quality and neutrality of the data so that they are free of biases, as these can be amplified in the models, perpetuating inequalities or prejudices.
Even if a dataset is technically open, its use in artificial intelligence models always raises some ethical implications. For example, it is necessary to avoid that personal or sensitive information is leaked or can be deduced from the results generated by the models, as this could cause damage to the privacy of individuals.
The issue of data attribution and intellectual property must also be taken into account. The use of open data in business models must address how the original creators of the data are recognised and adequately compensated so that incentives for creators continue to exist.
Open data is the engine that drives the amazing capabilities of language models, both SLM and LLM. While the SLMs stand out for their efficiency and accessibility, the LLMs open doors to advanced applications that not long ago seemed impossible. However, the path towards developing more capable, but also more sustainable and representative models depends to a large extent on how we manage and exploit open data.
Contenido elaborado por Jose Luis Marín, Senior Consultant in Data, Strategy, Innovation & Digitalization. Los contenidos y los puntos de vista reflejados en esta publicación son responsabilidad exclusiva de su autor.